5. Consistency We cannot always achieve unbiasedness of
12 Slides210.00 KB
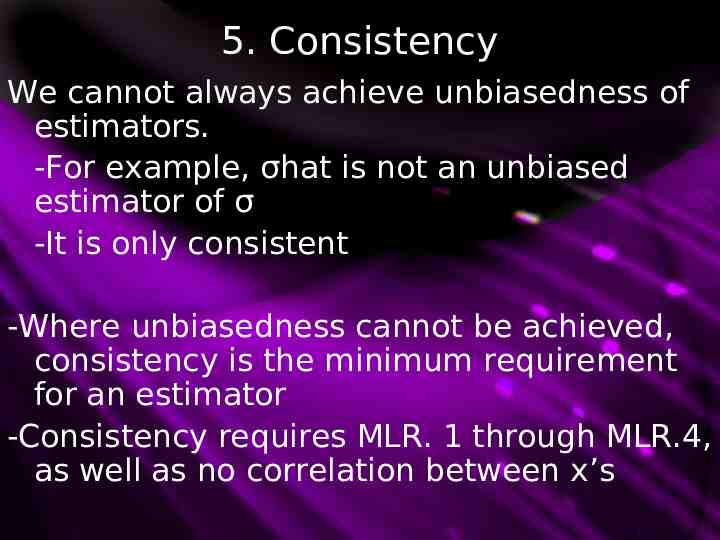
5. Consistency We cannot always achieve unbiasedness of estimators. -For example, σhat is not an unbiased estimator of σ -It is only consistent -Where unbiasedness cannot be achieved, consistency is the minimum requirement for an estimator -Consistency requires MLR. 1 through MLR.4, as well as no correlation between x’s

5. Intuitive Consistency While the actual proof of consistency is complicated, it can be intuitively explained -Each sample of n observations produces a Bjhat with a given distribution -MLR. 1 through MLR. 4 cause this Bjhat to be unbiased with mean Bj -If the estimator is consistent, as n increases the distribution becomes more tightly distributed around Bj -as n tends to infinity, Bjhat’s distribution collapses to Bj

5. Empirical Consistency In general, If obtaining more data DOES NOT get us closer to our parameter of interest We are using a poor (inconsistent) estimator. -Fortunately, the same assumptions imply unbiasedness and consistency:

Theorem 5.1 (Consistency of OLS) Under assumptions MLR. 1 through MLR. 4, the OLS estimator Bjhat is consistent for Bj for all j 0, 1, ,k.

Theorem 5.1 Notes While a general proof of this theorem requires matrix algebra, the single independent variable case can be proved from our B1hat estimator: ˆ1 (x (x ˆ1 1 i1 x1 ) y i i1 1 x1 ) 2 (x n 1 (x n i1 i1 (5.2) x1 )u i x1 ) 2 Which uses the fact that yi B0 B1xi1 u1 and previously seen algebraic properties

Theorem 5.1 Notes Using the law of large numbers, the numerator and denominator converge in probability to the population quantities Cov(x 1,u) and Var(x1) -Since Var(x1) 0 (MLR.3), we can use probability limits (Appendix C) to conclude: plim ˆ1 1 Cov(x1 , u) Var ( x1 ) (5.3) plim ˆ (since Cov(x , u ) 0) 1 1 1 Note that MLR.4, which assumes x 1 and u aren’t correlated, is essential to the above -Technically, Var(x1) and Var(u) should also be less than infinity

5. Correlation and Inconsistency -If MLR. 4 fails, consistency fails -that is, correlation between u and ANY x generally causes all OLS estimators to be inconsistent -”if the error is correlated with any of the independent variables, then OLS is biased and inconsistent” -in the simple regression case, the INCONSISTENCY in B 1hat (or ASYMPTOTIC BIAS) is: plim ˆ1 1 Cov(x1 , u) Var ( x1 ) (5.4)

5. Correlation and Inconsistency -Since variance is always positive, the sign of inconsistency depends on the sign of covariance -If the covariance is small compared to the variance, the inconsistency is negligible -However we can’t estimate this covariance as u is unobserved

5. Correlation and Inconsistency Consider the following true model: y 0 1 x1 2 x2 v Where we satisfy MLR.1 through MLR.4 (v has a zero mean and is uncorrelated with x1 and x2) -By Theorem 5.1 our OLS estimators (B jhat) are consistent -If we omit x2 and run an OLS regression, then u B 2x2 v and plim 1 1 2 1 (5.5) Cov ( x1 , x2 ) 1 (5.6) Var ( x1 )

5. Correlation and Inconsistency Practically, inconsistency can be viewed the same as bias -Inconsistency deals with population covariance and variance -Bias deals with sample covariance and variance -If x1 and x2 are uncorrelated, the delta1 0 and B1tilde is consistent (but not necessarily unbiased)

5. Inconsistency -The direction of inconsistency can be calculated using the same table as bias: Corr(x1,x2) 0 Corr(x1,x2) 0 B2hat 0 Positive Bias Negative Bias B2hat 0 Negative Bias Positive Bias

5. Inconsistency Notes If OLS is inconsistent, adding observations does not fix it -in fact, increasing sample size makes the problem worse -In the k regressor case, correlation between one x variable and u generally makes ALL coefficient estimators inconsistent -The one exception is when xj is correlated with u but ALL other variables are uncorrelated with both xj and u -Here only Bjhat is inconsistent