Data Quality Conceptual Framework and Dimensions Name, Data for Impact
14 Slides828.46 KB
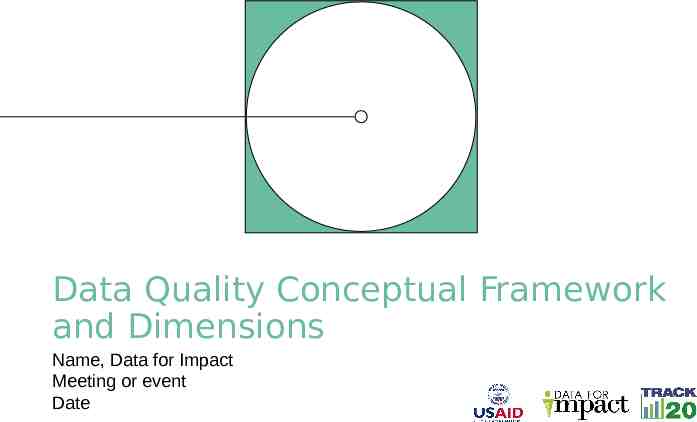
Data Quality Conceptual Framework and Dimensions Name, Data for Impact Meeting or event Date

Objectives: Understand the data quality conceptual framework tailored for FP. Define the dimensions of data quality. Identify the steps for assessing, improving, and maintaining data quality. Understand why data quality is important for the data use and decision-making process.

A Conceptual Framework for FP Data Quality INFORMATION SYSTEMS DECISIONS Interpretability of FP data signals Systems that incentivise data quality with data availability Analytics that inform at the level and the frequency needed TARGETED DATA QUALITY REVIEWS Rationalized indicators focusing on programme priorities Valid measurement of FP indicators and concepts Robust systems checks to flag data entry errors and outliers Automated Feedback loops Systematic Approach to Monitoring Data Quality in Family Planning Curriculum and Training on applying standardized methods- National Leveraging systems investments over ad-hoc supportive supervision Efficient use of Monitoring Resources focusing on issues/areas of highest need PEOPLE Dedicated staff time at National levels for FP Data Routine mechanisms that include FP Data Quality Review Capacity to identify and prioritize for data quality action

Improving Data Quality in FP: Tools 1. Service Statistics to Estimated Modern Use (SS to EMU) tool: Excelbased tool that is typically applied at the national level and can be applied subnationally. Value: Can identify where problems in data quality are occurring and for which methods. Value: Currently being used for FP2020 by Track20-supported countries and government technical staff. 2. DHIS2 FP Generic Module: Comprehensive environment to review data quality and its linkage to performance. Value: Contains the SS to EMU tool approach. Value: Available to embed in the DHIS2. Value: Strengthens the health management information system (HMIS) and reduces the resource burden for data quality. 3. RDQA for assessing sources of poor data quality: Facility-based tool Value: Standardized approach to routine data quality at the facility level.

Leveraging opportunities in existing tools 1. 2. 3. 4. These tools provide information at different levels and depths. SS to EMU tool and the FP Generic Module can identify where in-depth reviews are most needed. RDQAs can provide indepth information on the nature and drivers of poor data quality. Combining both allows programs to efficiently target scarce data quality resources AND improve outcomes.

How to describe the way that the two tools interact along the continuum of data quality assessment

Data Quality Conceptual Framework Using RDQA

Data Quality Dimensions for Systems and RDQA Application DIMENSIONS RDQAs cannot occur everywhere given resource constraints. Data quality should be applied to the FP information system to ensure that data elements and indicators in the system can produce quality data as constructed. The same dimensions are used during a RDQA. Accuracy - they measure what they are intended to measure Completeness - collected comprehensively Reliability - repeated measurements using the same procedures get the same results Timeliness - up-to-date & available on time Confidentiality - clients’ data are not disseminated Precision - have sufficient detail to use for decision making Integrity - protected from bias or manipulation Validity - considered accurate—they measure what they are intended to measure Timeliness - means that the data are sufficiently current and frequent to inform management’s decision making. They are received by the established deadline. Completeness - Comprehensive data collection; percentage of all fields in the data collection form filled in; percentage of all expected reports received.

Data Quality Dimensions Validity o Considered accurate—they measure what they are intended to measure. Timeliness o Data are sufficiently current and frequent to inform management’s decision making. o They are received by the established deadline. Completeness o Comprehensive data collection o Percentage of all fields on the data collection form filled in. o Percentage of all expected reports actually received.

Factors that affect data quality at all levels of the information system Key Steps to Guarantee Data Quality Standard data collection tools and reporting forms Steps addressing quality challenges Specific reporting timelines Description of roles and responsibilities Storage policy that allows for retrieval of data Functioning information systems, including FP Skilled HMIS staff at the different levels of the health system Clear definitions of indicators Documented data review procedures

Good FP data are needed to: Plan, develop, and maintain FP services. Influence decision making on the quality of the FP service and care continuum. Evaluate the performance of FP services over time and by location. Consider future needs of the FP program and interventions. Monitor FP service achievements against standards and targets. Improve the use of FP services in a community. Determine staffing requirements and plan FP services.

Group Exercise Identify where RDQA should take place Comparing mCPR to EMU from commodities and visits 250% 200% 150% 100% 50% 0% 2010 2011 2012 2013 2014 2015 EMU (commodities) EMU (visits) mCPR (AW) from surveys These bar graphs compare the distribution of users according to HMIS data and for the same year from a DHS survey. What does this tell you about which methods need more review? What do you notice immediately about trends from commodities data? How will you determine if the trend and spike in 2014 reflect accuracy?

Group Exercise Once Geographies and Methods Are Identified ‒ FacilityLevel RDQA Scenarios: Angela visited a health facility after having unprotected intercourse with her boyfriend, fearing possible pregnancy. She requested counseling and advice from a FP provider. After the counseling session, the provider and Angela agree that she should take an emergency contraceptive pill. Paul, a young boy schooling and residing in Ajegunle, Lagos asked for a condom from a FP provider. He was referred to the STI unit and received 12 condoms. Angela’s boyfriend named Adamu visited the same facility that Angela visited 30 minutes after her to request an emergency contraceptive pill. He told the FP provider that he had unprotected sex with his girlfriend and he was not ready to be a father. The provider gave Adamu an emergency contraceptive pill to give to his girlfriend. During the reporting period, the data manager was asked to give a report of FP users at the facility. From the scenarios above: o How many FP users should the data manager report for the period? o How did you determine the number of FP users?

This presentation was produced with the support of the United States Agency for International Development (USAID) under the terms of the Data for Impact (D4I) associate award 7200AA18LA00008, which is implemented by the Carolina Population Center at the University of North Carolina at Chapel Hill, in partnership with Palladium International, LLC; ICF Macro, Inc.; John Snow, Inc.; and Tulane University. The views expressed in this publication do not necessarily reflect the views of USAID or the United States government. www.data4impactproject.org