How Neurons Do Integrals Mark Goldman
21 Slides4.05 MB
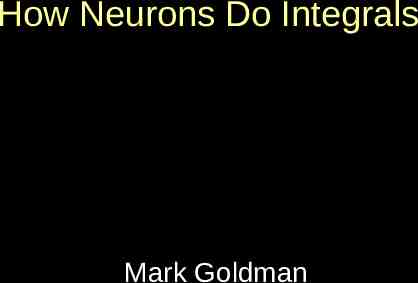
How Neurons Do Integrals Mark Goldman
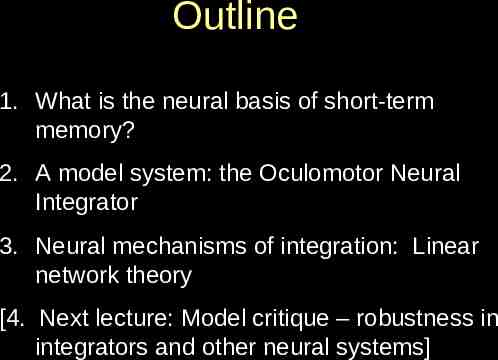
Outline 1. What is the neural basis of short-term memory? 2. A model system: the Oculomotor Neural Integrator 3. Neural mechanisms of integration: Linear network theory [4. Next lecture: Model critique – robustness in integrators and other neural systems]
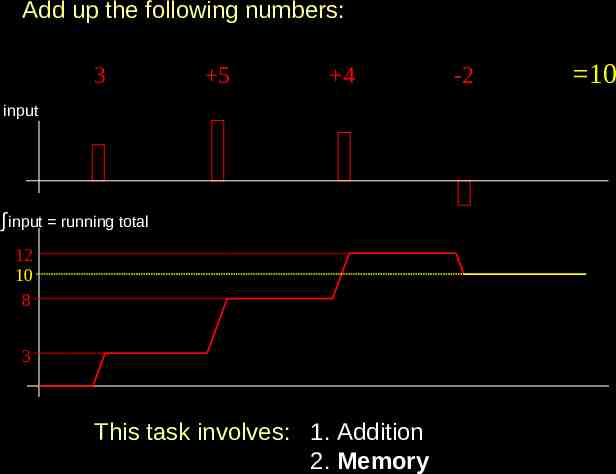
Add up the following numbers: 3 5 4 input input running total 12 10 8 3 This task involves: 1. Addition 2. Memory -2 10
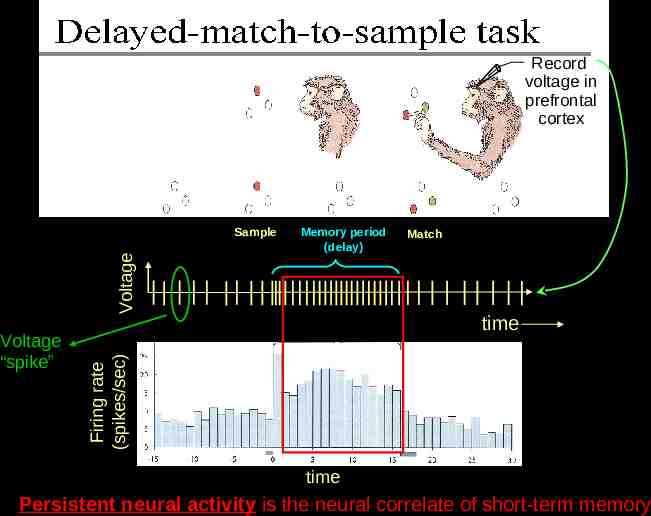
Record voltage in prefrontal cortex Voltage “spike” Memory period (delay) Match time Firing rate (spikes/sec) Voltage Sample time Persistent neural activity is the neural correlate of short-term memory
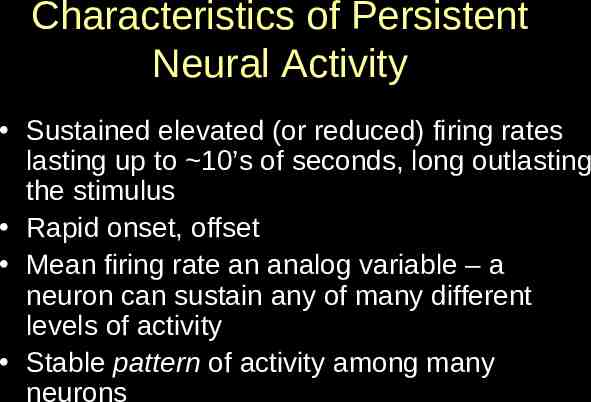
Characteristics of Persistent Neural Activity Sustained elevated (or reduced) firing rates lasting up to 10’s of seconds, long outlasting the stimulus Rapid onset, offset Mean firing rate an analog variable – a neuron can sustain any of many different levels of activity Stable pattern of activity among many neurons
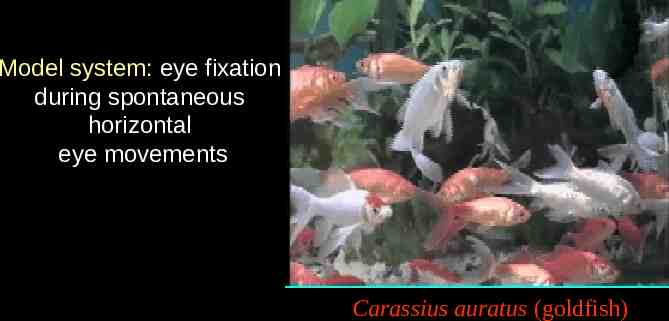
Model system: eye fixation during spontaneous horizontal eye movements Carassius auratus (goldfish)
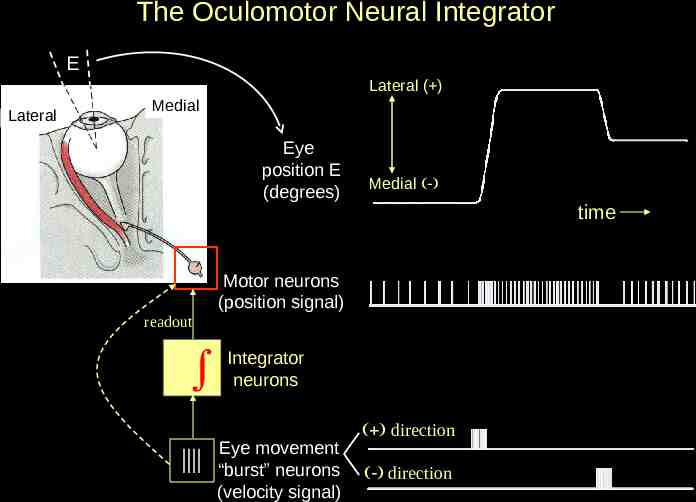
The Oculomotor Neural Integrator E Lateral ( ) Lateral Medial Eye position E (degrees) Medial time Motor neurons (position signal) readout Integrator neurons Eye movement “burst” neurons (velocity signal) direction direction
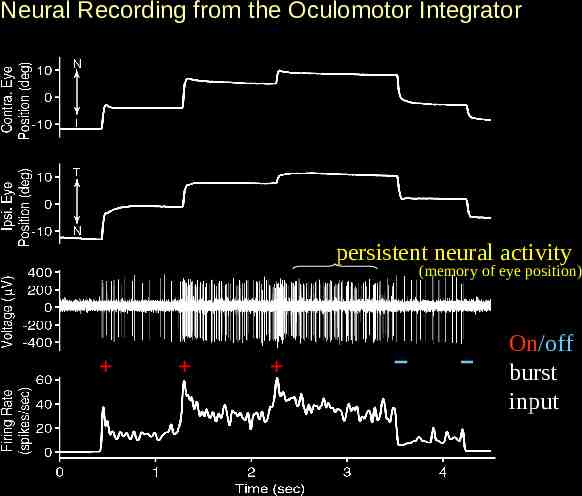
Neural Recording from the Oculomotor Integrator persistent neural activity (memory of eye position) On/off burst input
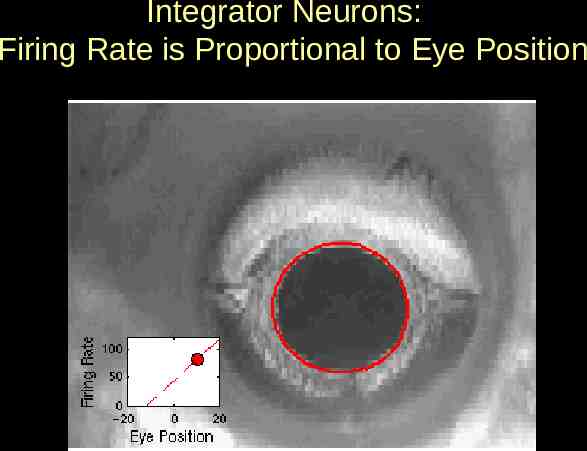
Integrator Neurons: Firing Rate is Proportional to Eye Position
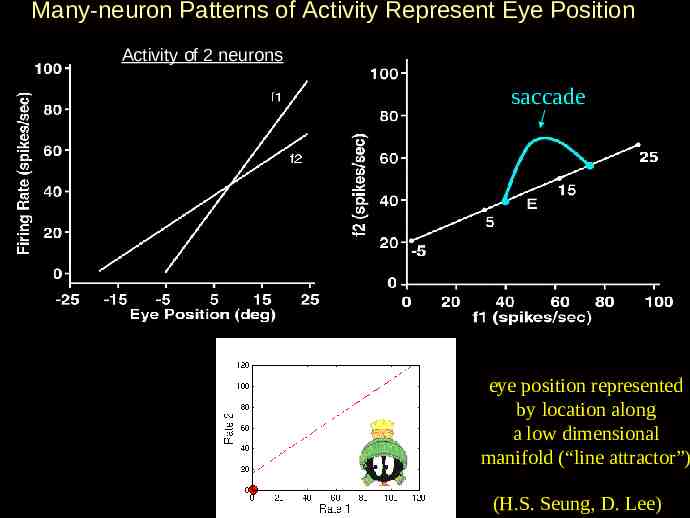
Many-neuron Patterns of Activity Represent Eye Position Activity of 2 neurons saccade eye position represented by location along a low dimensional manifold (“line attractor”) (H.S. Seung, D. Lee)
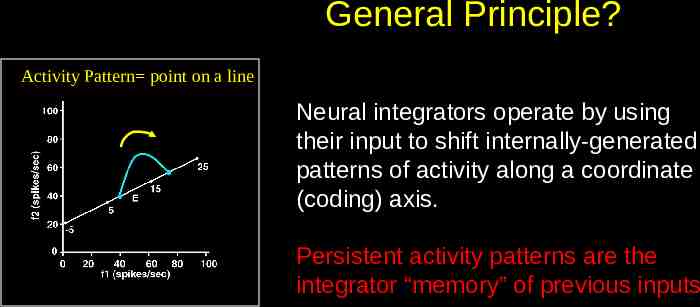
General Principle? Activity Pattern point on a line Neural integrators operate by using their input to shift internally-generated patterns of activity along a coordinate (coding) axis. Persistent activity patterns are the integrator “memory” of previous inputs

Mechanisms and Models of Persistent Neural Activity
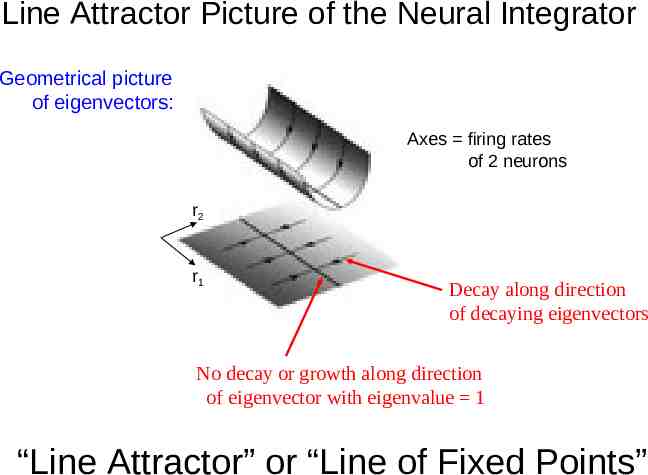
Line Attractor Picture of the Neural Integrator Geometrical picture of eigenvectors: Axes firing rates of 2 neurons r2 r1 Decay along direction of decaying eigenvectors No decay or growth along direction of eigenvector with eigenvalue 1 “Line Attractor” or “Line of Fixed Points”
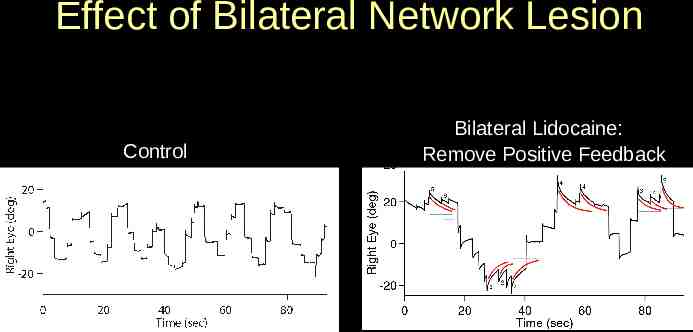
Effect of Bilateral Network Lesion Control Bilateral Lidocaine: Remove Positive Feedback
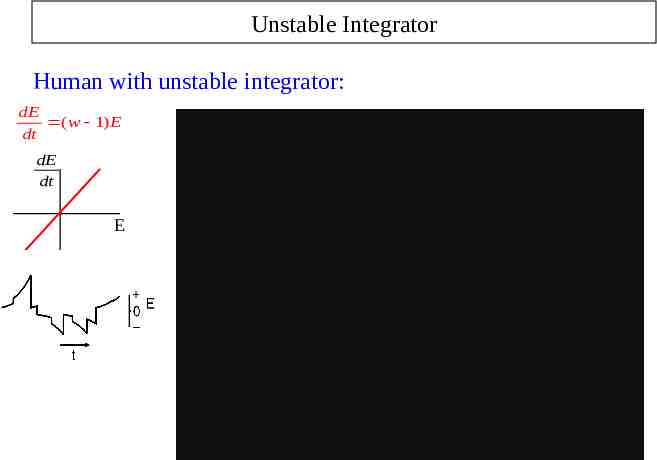
Unstable Integrator Human with unstable integrator: dE ( w 1) E dt dE dt E
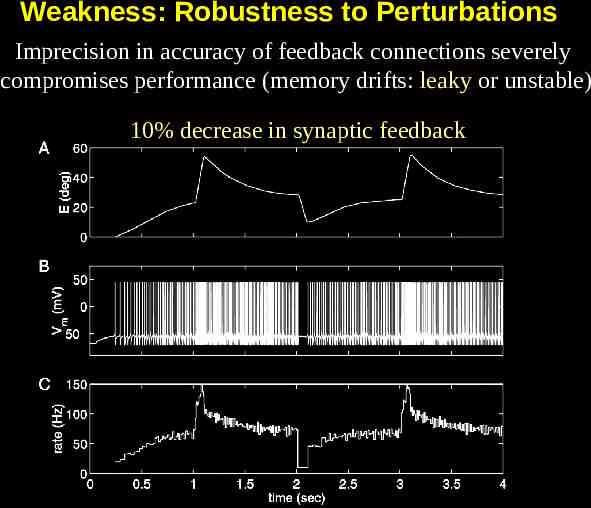
Weakness: Robustness to Perturbations Imprecision in accuracy of feedback connections severely compromises performance (memory drifts: leaky or unstable) 10% decrease in synaptic feedback
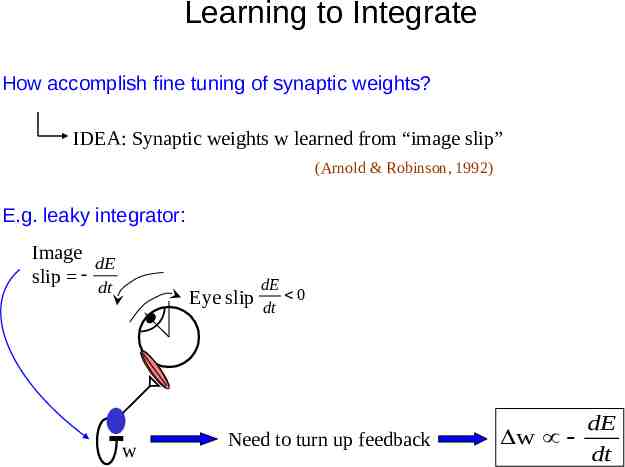
Learning to Integrate How accomplish fine tuning of synaptic weights? IDEA: Synaptic weights w learned from “image slip” (Arnold & Robinson, 1992) E.g. leaky integrator: Image dE slip dE dt Eye slip dt 0 w Need to turn up feedback w dE dt
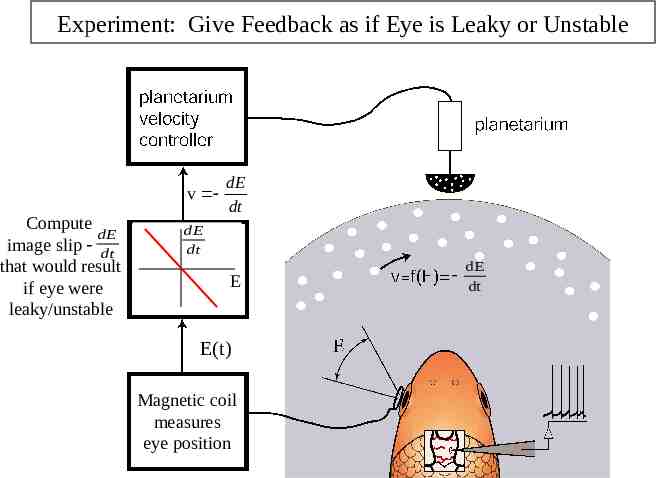
Experiment: Give Feedback as if Eye is Leaky or Unstable v Compute dE image slip dt that would result if eye were leaky/unstable dE dt dE dt E E(t) Magnetic coil measures eye position dE dt
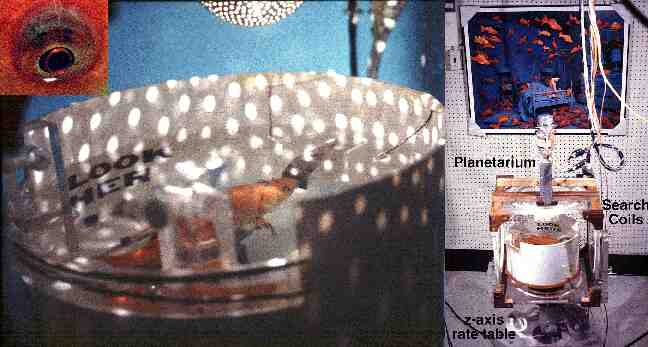
Integrator Learns to Compensate for Leak/Instability! Control (in dark): Give feedback as if unstable Leaky: Give feedback as if leaky Unstable:
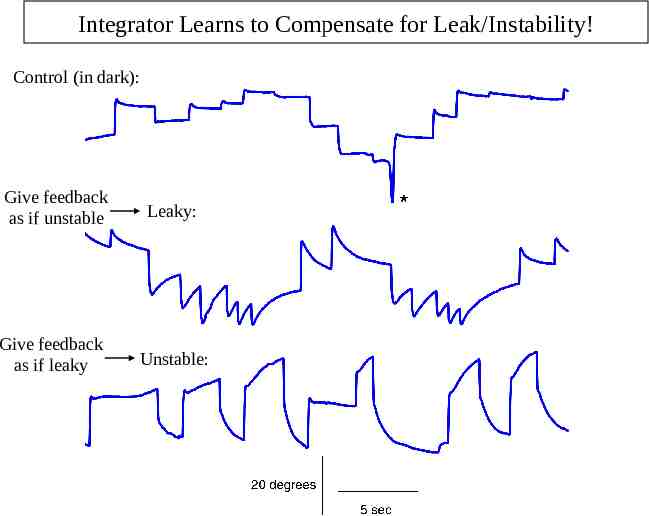
Summary Persistent neural activity: activity which outlasts a transient stimulus Neural integrator: outputs the mathematical integral of its inputs -in the absence of input, maintains persistent neural activity Oculomotor neural integrator: -converts eye-velocity encoding inputs to eye position outputs -Mechanism: -Anatomy and physiology suggest network contribution -Issue of Robustness: Simple models are less robust to perturbations than the real system But: -Plasticity may keep the system tuned on slow time scales -On faster time scales, intrinsic cellular processes may provide a “friction-like” slowing of network decay
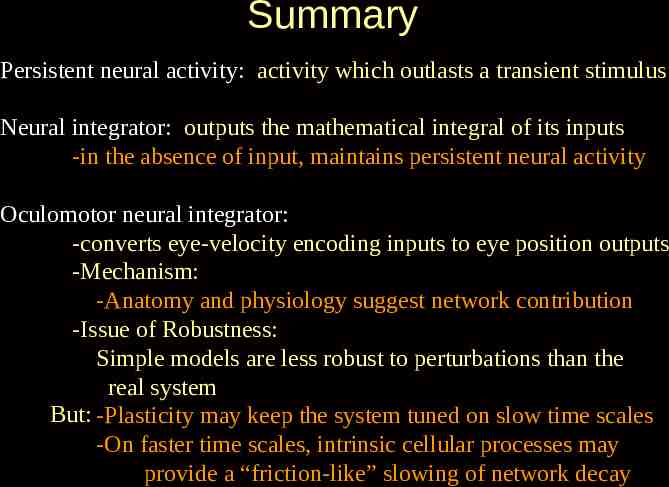