Analysis of Data with Missing Values Chakra Budhathoki, PhD
27 Slides612.87 KB
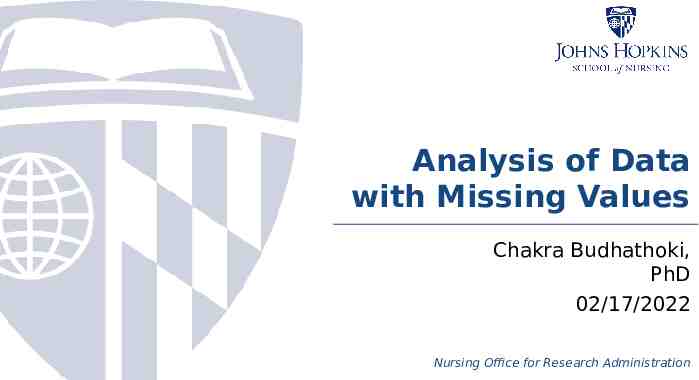
Analysis of Data with Missing Values Chakra Budhathoki, PhD 02/17/2022 Nursing Office for Research Administration
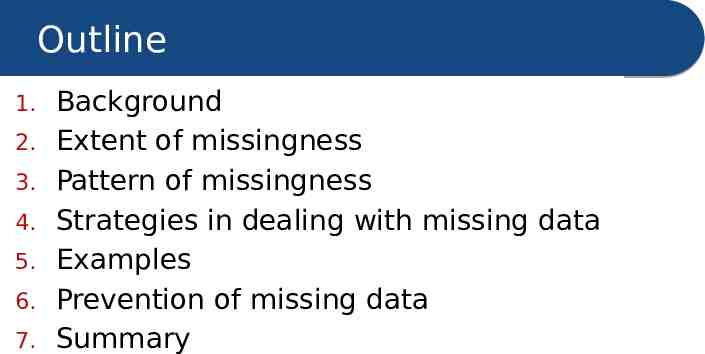
Outline 1. 2. 3. 4. 5. 6. 7. Background Extent of missingness Pattern of missingness Strategies in dealing with missing data Examples Prevention of missing data Summary
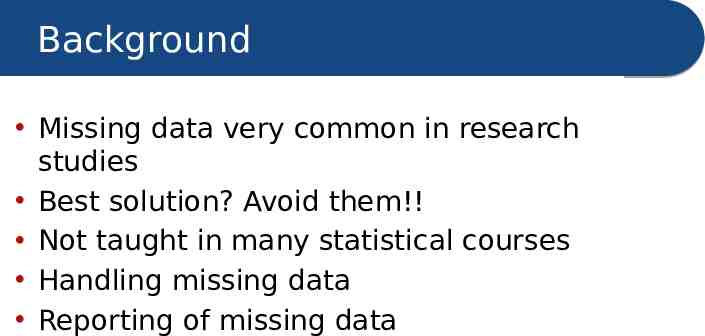
Background Missing data very common in research studies Best solution? Avoid them!! Not taught in many statistical courses Handling missing data Reporting of missing data
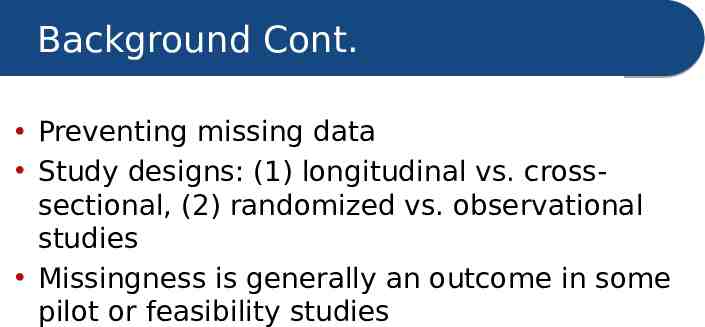
Background Cont. Preventing missing data Study designs: (1) longitudinal vs. crosssectional, (2) randomized vs. observational studies Missingness is generally an outcome in some pilot or feasibility studies
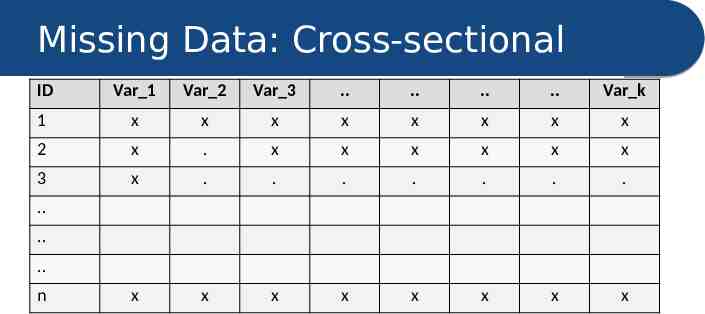
Missing Data: Cross-sectional ID Var 1 Var 2 Var 3 . . . . Var k 1 x x x x x x x x 2 x . x x x x x x 3 x . . . . . . . x x x x x x x x . . . n
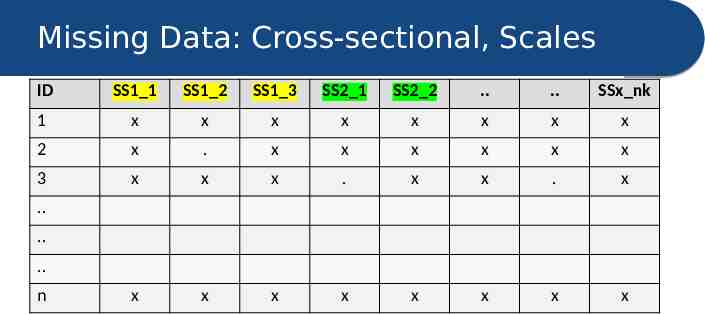
Missing Data: Cross-sectional, Scales ID SS1 1 SS1 2 SS1 3 SS2 1 SS2 2 . . SSx nk 1 x x x x x x x x 2 x . x x x x x x 3 x x x . x x . x x x x x x x x x . . . n
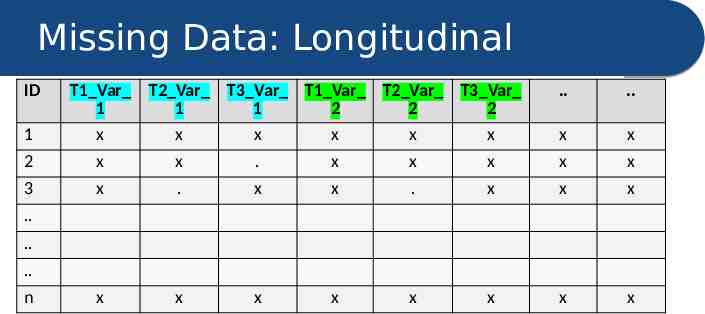
Missing Data: Longitudinal ID 1 T1 Var 1 x T2 Var T3 Var T1 Var T2 Var T3 Var 1 1 2 2 2 x x x x x . . x x 2 x x . x x x x x 3 x . x x . x x x x x x x x x x x . . . n
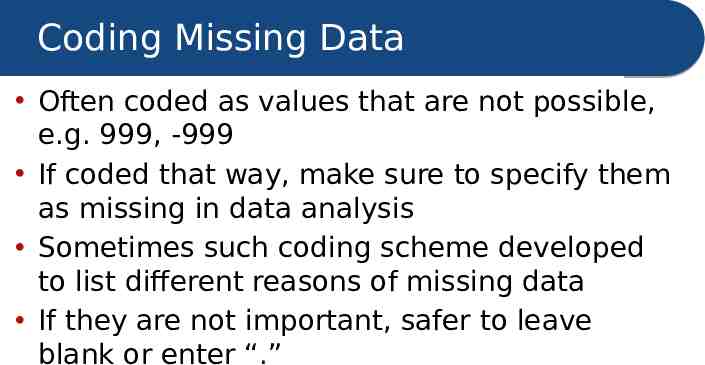
Coding Missing Data Often coded as values that are not possible, e.g. 999, -999 If coded that way, make sure to specify them as missing in data analysis Sometimes such coding scheme developed to list different reasons of missing data If they are not important, safer to leave blank or enter “.”
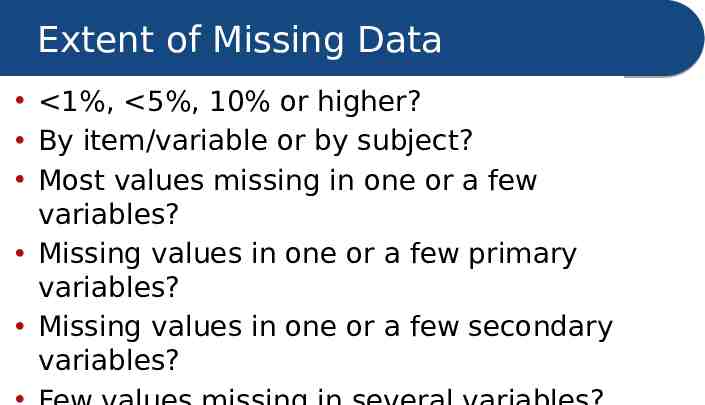
Extent of Missing Data 1%, 5%, 10% or higher? By item/variable or by subject? Most values missing in one or a few variables? Missing values in one or a few primary variables? Missing values in one or a few secondary variables?
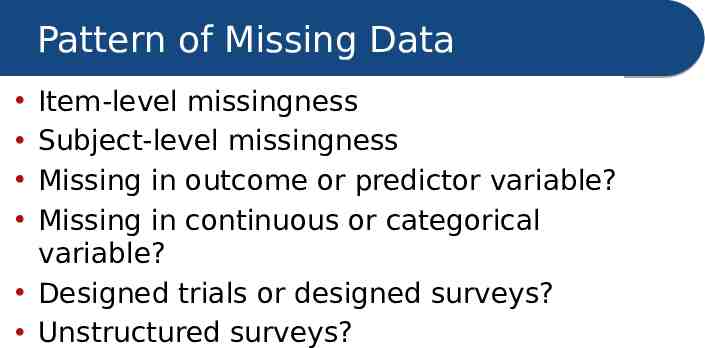
Pattern of Missing Data Item-level missingness Subject-level missingness Missing in outcome or predictor variable? Missing in continuous or categorical variable? Designed trials or designed surveys? Unstructured surveys?
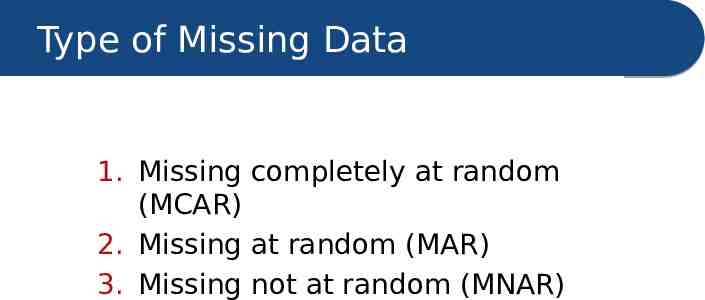
Type of Missing Data 1. Missing completely at random (MCAR) 2. Missing at random (MAR) 3. Missing not at random (MNAR)
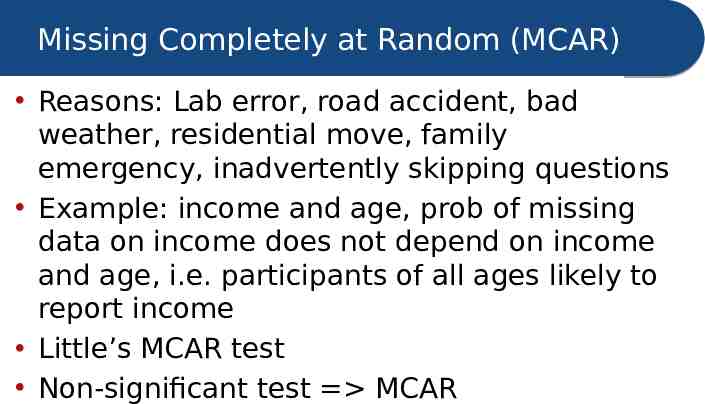
Missing Completely at Random (MCAR) Reasons: Lab error, road accident, bad weather, residential move, family emergency, inadvertently skipping questions Example: income and age, prob of missing data on income does not depend on income and age, i.e. participants of all ages likely to report income Little’s MCAR test Non-significant test MCAR
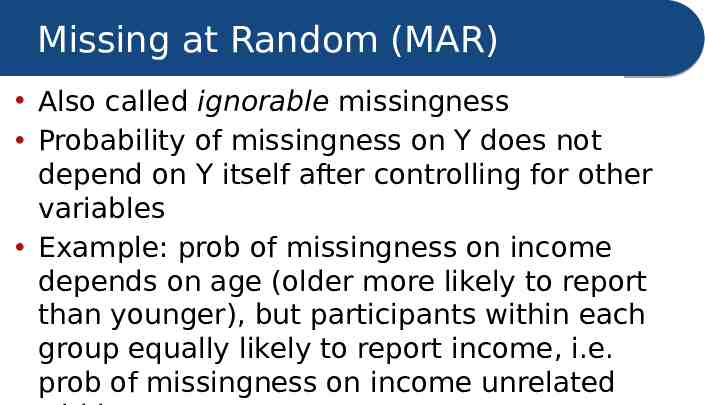
Missing at Random (MAR) Also called ignorable missingness Probability of missingness on Y does not depend on Y itself after controlling for other variables Example: prob of missingness on income depends on age (older more likely to report than younger), but participants within each group equally likely to report income, i.e. prob of missingness on income unrelated
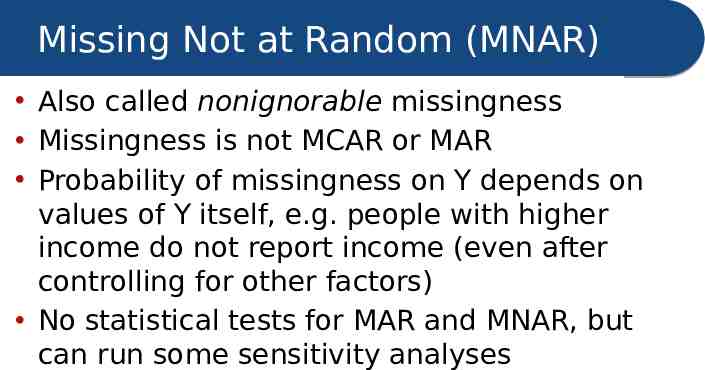
Missing Not at Random (MNAR) Also called nonignorable missingness Missingness is not MCAR or MAR Probability of missingness on Y depends on values of Y itself, e.g. people with higher income do not report income (even after controlling for other factors) No statistical tests for MAR and MNAR, but can run some sensitivity analyses
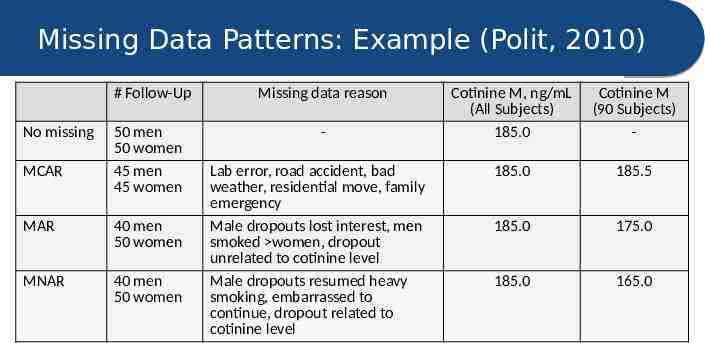
Missing Data Patterns: Example (Polit, 2010) No missing MCAR # Follow-Up Missing data reason 50 men 50 women 45 men 45 women - MAR 40 men 50 women MNAR 40 men 50 women Lab error, road accident, bad weather, residential move, family emergency Male dropouts lost interest, men smoked women, dropout unrelated to cotinine level Male dropouts resumed heavy smoking, embarrassed to continue, dropout related to cotinine level Cotinine M, ng/mL (All Subjects) 185.0 Cotinine M (90 Subjects) - 185.0 185.5 185.0 175.0 185.0 165.0
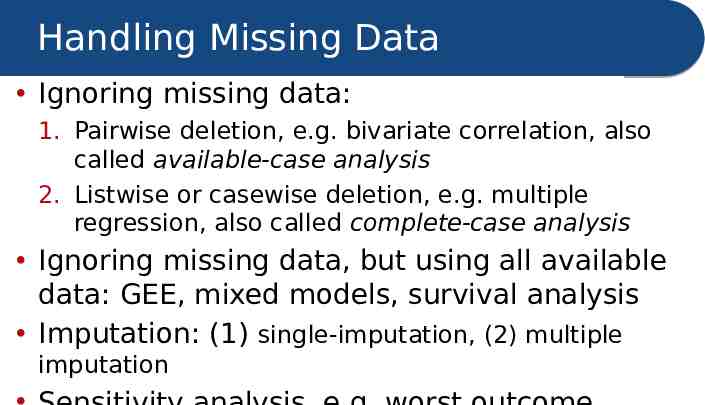
Handling Missing Data Ignoring missing data: 1. Pairwise deletion, e.g. bivariate correlation, also called available-case analysis 2. Listwise or casewise deletion, e.g. multiple regression, also called complete-case analysis Ignoring missing data, but using all available data: GEE, mixed models, survival analysis Imputation: (1) single-imputation, (2) multiple imputation
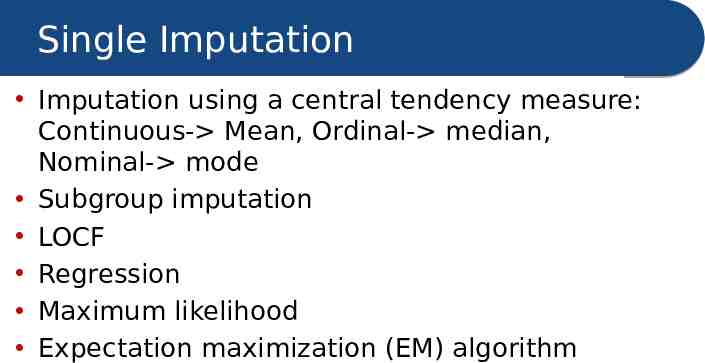
Single Imputation Imputation using a central tendency measure: Continuous- Mean, Ordinal- median, Nominal- mode Subgroup imputation LOCF Regression Maximum likelihood Expectation maximization (EM) algorithm
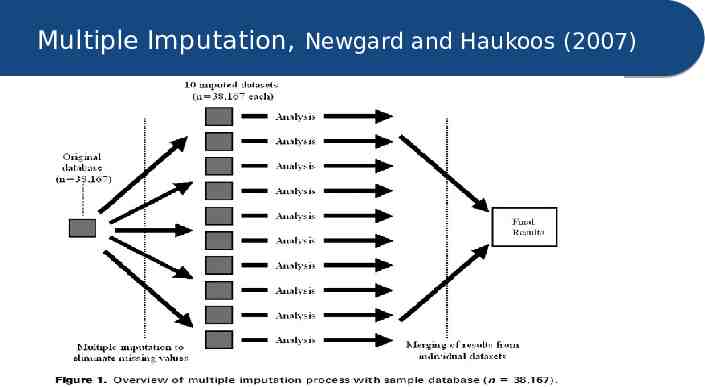
Multiple Imputation, Newgard and Haukoos (2007)
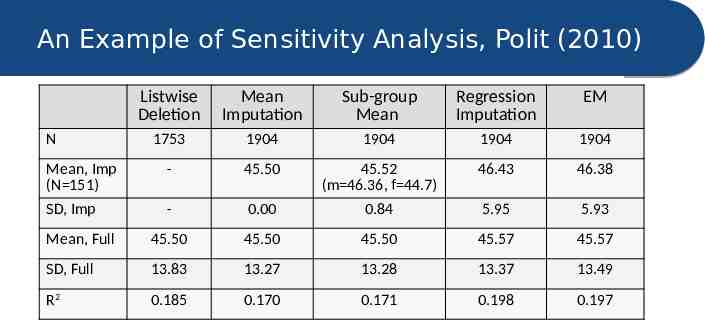
An Example of Sensitivity Analysis, Polit (2010) Listwise Deletion Mean Imputation Sub-group Mean Regression Imputation EM 1753 1904 1904 1904 1904 Mean, Imp (N 151) SD, Imp - 45.50 46.43 46.38 - 0.00 45.52 (m 46.36, f 44.7) 0.84 5.95 5.93 Mean, Full 45.50 45.50 45.50 45.57 45.57 SD, Full 13.83 13.27 13.28 13.37 13.49 R2 0.185 0.170 0.171 0.198 0.197 N
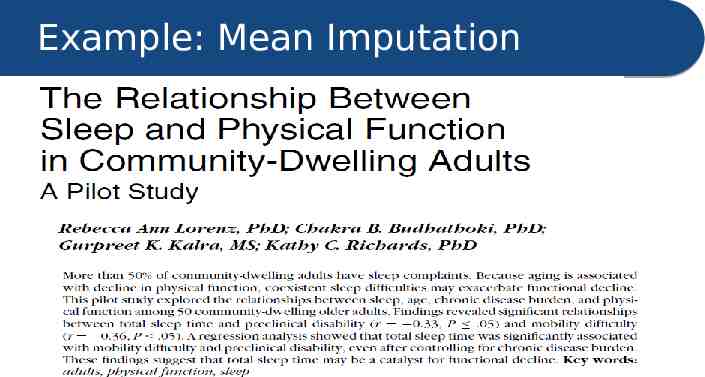
Example: Mean Imputation
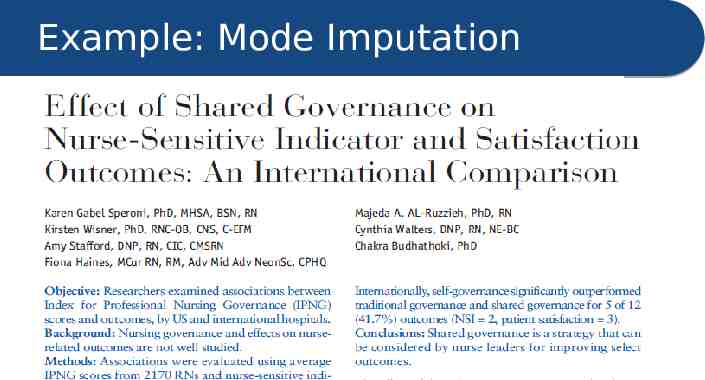
Example: Mode Imputation
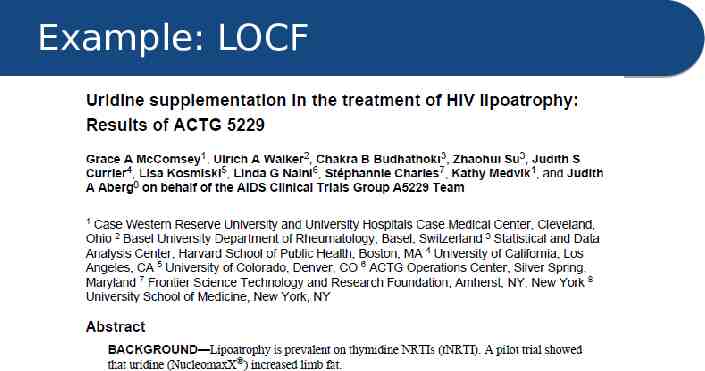
Example: LOCF
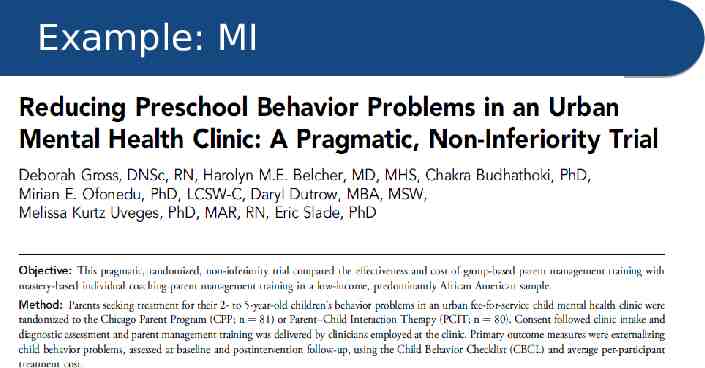
Example: MI
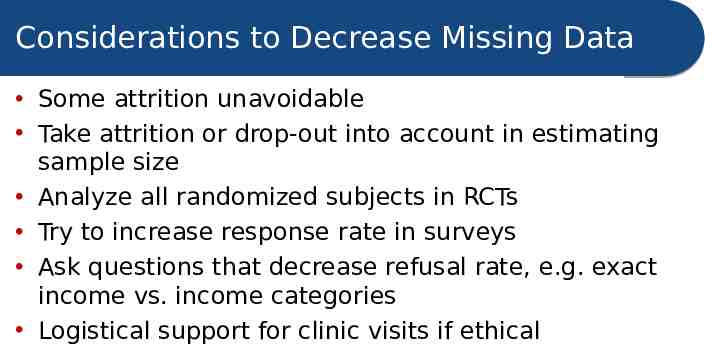
Considerations to Decrease Missing Data Some attrition unavoidable Take attrition or drop-out into account in estimating sample size Analyze all randomized subjects in RCTs Try to increase response rate in surveys Ask questions that decrease refusal rate, e.g. exact income vs. income categories Logistical support for clinic visits if ethical
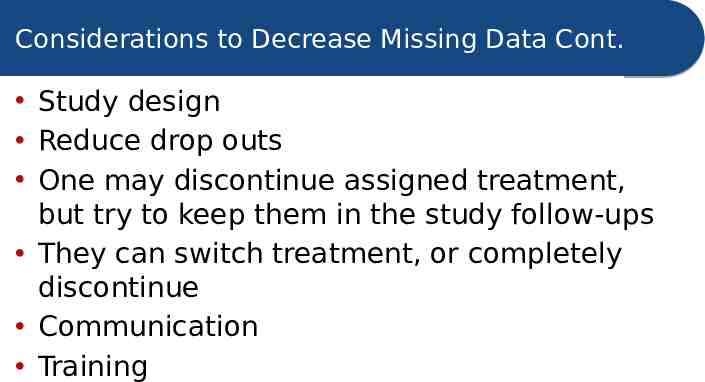
Considerations to Decrease Missing Data Cont. Study design Reduce drop outs One may discontinue assigned treatment, but try to keep them in the study follow-ups They can switch treatment, or completely discontinue Communication Training
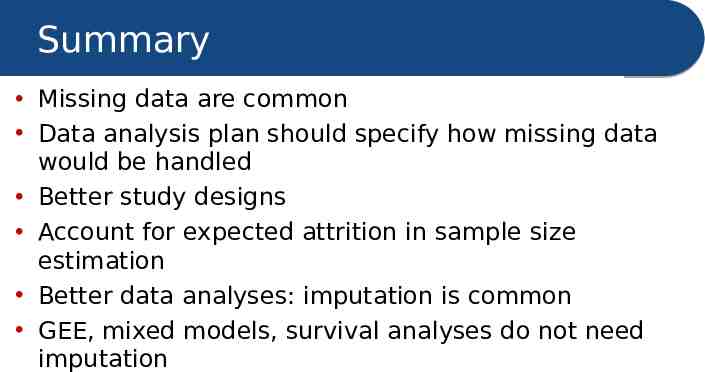
Summary Missing data are common Data analysis plan should specify how missing data would be handled Better study designs Account for expected attrition in sample size estimation Better data analyses: imputation is common GEE, mixed models, survival analyses do not need imputation
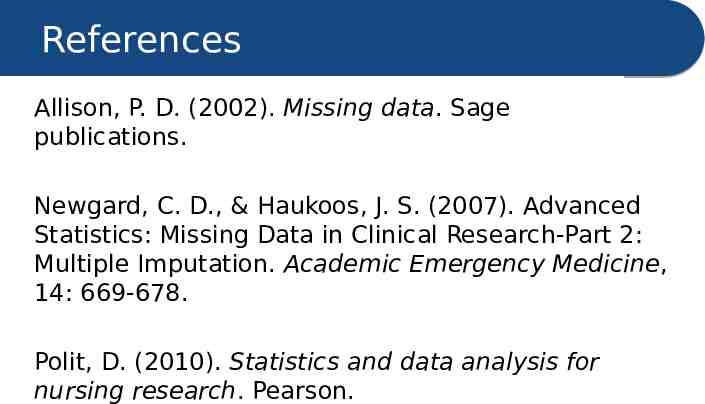
References Allison, P. D. (2002). Missing data. Sage publications. Newgard, C. D., & Haukoos, J. S. (2007). Advanced Statistics: Missing Data in Clinical Research-Part 2: Multiple Imputation. Academic Emergency Medicine, 14: 669-678. Polit, D. (2010). Statistics and data analysis for nursing research. Pearson.