Life Cycle Data Mining Gregg Vesonder Jon Wright Tamparni Dasu
25 Slides77.00 KB
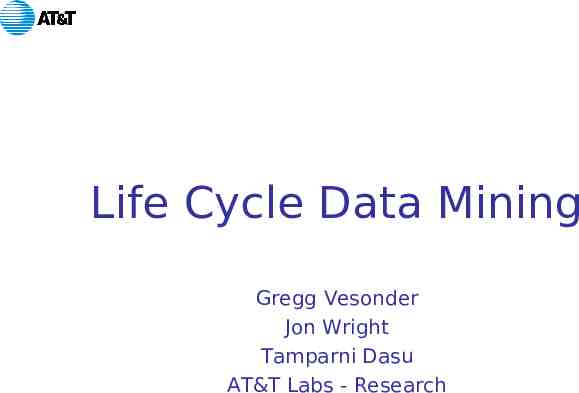
Life Cycle Data Mining Gregg Vesonder Jon Wright Tamparni Dasu AT&T Labs - Research

Roadmap Bouillabaisse vs Stone Soup The Life Cycle On the Data Mise en place Preservation Case Studies - Some ESs, KDD Paper Data Mining Gastronomique 01/23/23 Vesonder, Wright, Dasu 2

So? Systems Approach Unique issues and combinations of issues – – – – – Mise en place [most all] runs are unique Data Quality is crucial Granularity Downstream systems Process issues – Knowledge engineering throughout – Verification and validation issues 01/23/23 Vesonder, Wright, Dasu 3

Bouillabaisse Data Mining Data exists in some repository/corpus Know the fields and relationships At least familiar with some domain Others have mined the data - community Reference efforts -- helps Verification (built system right) and Validation (built right system) World Wide Telescope - Jim Gray 01/23/23 Vesonder, Wright, Dasu 4

Stone Soup Data Mining A Fable in many parts The data is not in one place, in fact it is in many places – Don’t know the quality – Don’t know what it means and there is no one source to discover it (multiple, conflicting experts - Brooks “never go to sea with two chronometers, go with one or three”) Data does not remain there - have to capture it -- usually on arcane systems 01/23/23 Vesonder, Wright, Dasu 5

Stone Soup -2 Once you get it - more experts, pilot runs (very much like Knowledge Engineering technique) – BTW it is in EBCDIC, described by COBOL copybooks, you’re running UNIX Discover you need other data to interpret it - back to previous page At this point it has been months - if lucky Time to formalize the collection process Did I mention the data is huge! Time to do some “data mining” - knowledge and quality Archiving issues - reproduction (depends on what is available and who contributes) 01/23/23 Vesonder, Wright, Dasu 6

Knowledge Engineering Technique (So old that it needs to be reprised) Knowledge Engineer becomes familiar with domain, architecture and operation KE meets with experts to understand operations and issues Team uses knowledge to create first (and subsequent) passes at working system Experts critique results, provide new knowledge and iterate on previous step until a satisfactory (or best possible) conclusion is achieved 01/23/23 Vesonder, Wright, Dasu 7

Stone Soup-3 About this time one of your feeds changes - actually it was several months ago Verification and validation throughout Preservation of data, summarized data, interim reports and techniques really time “encapsules” 01/23/23 Vesonder, Wright, Dasu 8

A View of the Space Data Quality “Data Mining” [Knowledge System *] Engineering Data Acquisition & Preparation 01/23/23 (mise en Data Preservation Vesonder, Wright, Dasu 9

A Rough Estimate of the Effort Of course the 10% can grow over time, but "Data Mining" All Else 01/23/23 Vesonder, Wright, Dasu 10

The Life Cycle Discover data needed - KE Get data/Establish Feed – Discover and perhaps get additional data to interpret data - KE – Verify & Validate feed – Assess data quality Discover Reference results for V & V (may be earlier) Prepare environment and Run Data V &V - KE (iterate - may take you to top again) Preserve environment and archive Continuously check “upstream” issues - improve data quality Usually there is increased level of understanding 01/23/23 Vesonder, Wright, Dasu 11

Knowledge Engineering (KE) Book Knowledge on topic sparse Parni on calls for months - patience to find knowledge nuggets – Finding appropriate expert but: Current project 50% of time on calls with Subject Matter Experts Experts Disagree - more conference calls Initial run - bridge knowledge gap other way Prep/Run time measured in large units 01/23/23 Vesonder, Wright, Dasu 12

Preservation No ready made archives Preserve data, software and comparisons – Data and meta data synchronized (e.g. time dependent) – Redundancy, security, . – Recoverability 01/23/23 Vesonder, Wright, Dasu 13

The Data Attributes (APOLOGIES - COULD NOT FIND PREDEFINED TAXONOMY) Single vs multiple streams Self contained -several ways Temporally based - several ways Accessible repository Reference implementation - testing, V&V Size Complexity (a work in progress, more to come) 01/23/23 Vesonder, Wright, Dasu 14

Mise en place “put in place” chopping, mincing, measurement, peeling, washing Significant planning activity to start a run – Data ready - off tape and accessible - could be N different feeds – Data verified – Sufficient system resources (disk, memory, ) – Consistent software builds Candidate for AI planning techniques, ES for monitoring run (insuring available disk resources, trapping failures, ) 01/23/23 Vesonder, Wright, Dasu 15

ACE experience Expert system for cable maintenance Specialized tools but not specialized environment - close to operations Quick studies on the domain - key factor Dealing with multiple experts Most (80 %) of the work was not ES 01/23/23 Vesonder, Wright, Dasu 16

KDD Paper Example Case study from KDD AI techniques addressing quality issues of the data Instance of our general methodology that can be used at every stage of the lifecycle - Knowledge Engineering based Spent a lifetime in multi hour conference calls 01/23/23 Vesonder, Wright, Dasu 17

Data Quality Dasu, Vesonder, Wright Common for operations databases to have 60-90% bad data Audits are used to detect errors for later correction Enlightened approach is to proactively prevent errors before they occur BUT the business operations rules for these databases are inaccurate and incomplete and acquiring it has challenges. The solution we presented was using Knowledge Engineering and Rule Based programming to capture and represent the data. 01/23/23 Vesonder, Wright, Dasu 18

Typical Project Characteristics Knowledge is available in a fragmentary way, often out of logical or operational sequence Expertise is split across organizations little incentive to cooperate Business rules change frequently Experts do not agree - inconsistent rules Project personnel change frequently Little project accountability in matrixed organizations 01/23/23 Vesonder, Wright, Dasu 19

Knowledge Engineering Knowledge Engineer becomes familiar with domain, architecture and operation KE meets with experts to understand operations and issues Team uses knowledge to create first (and subsequent) passes at rules Experts critique results, provide new knowledge and iterate on previous step until a satisfactory (or best possible) conclusion is achieved 01/23/23 Vesonder, Wright, Dasu 20

Quality Case Study 20 experts - a challenge Original in SAS Rule conversion focused knowledge in meaningful, manipulatable chunks Data quality engineer of present and future will need techniques to capture, vet and deploy knowledge of the data, process and necessary continuous audits and do this at scale. 01/23/23 Vesonder, Wright, Dasu 21

Working Memory Rule Base (Bus. Ops Database) (Bus. Rules/Data Specs) Data Records Match Database Modifications Act Conflict Set (Candidate Rules) Selected Rule Conflict Resolution (Assign Priority) Interpreter 01/23/23 Vesonder, Wright, Dasu 22

Mise en place and Planning Planning algorithms, means-ends analysis to do cutting and chopping – – – – – Check for and Secure resources Assemble data Schedule jobs Monitor run Assemble output -- distributed computing – Flag results 01/23/23 Vesonder, Wright, Dasu 23

Data Mining Gastronomique Data Quality - see Parni & Ted book reference AI Techniques: – Planning - especially for Mise en place – Expert Systems - Rule base/Agent systems for monitoring/quality Also use Ganglia and other tools – KE at most points 01/23/23 Vesonder, Wright, Dasu 24

Conclusions Provider a broader view of what constitutes data mining Process orientation - addresses complete system development – Sometimes the data isn’t on the web, in a corpus or on a CD – Quality issues Mise en place a big issue, since each run is special AI as one approach to the issues Much more coming 01/23/23 Vesonder, Wright, Dasu 25