Investigative analytics and derived data The example of
20 Slides473.00 KB

Investigative analytics and derived data The example of customer acquisition & retention Curt A. Monash, Ph.D. President, Monash Research Editor, DBMS2 http://www.monash.com http://www.DBMS2.com
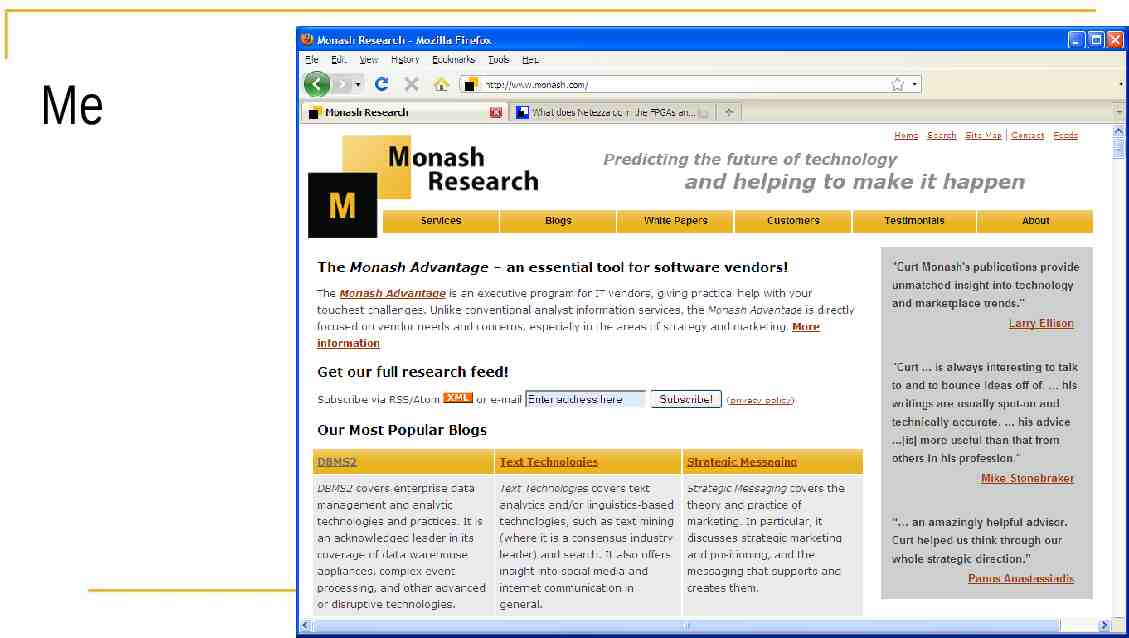
Me
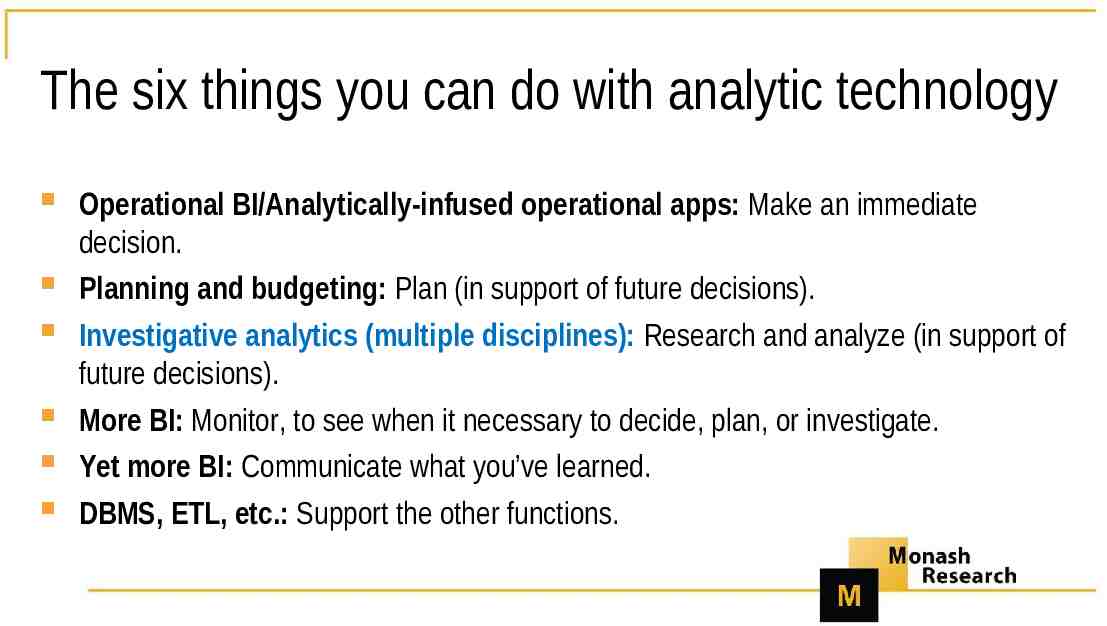
The six things you can do with analytic technology Operational BI/Analytically-infused operational apps: Make an immediate decision. Planning and budgeting: Plan (in support of future decisions). Investigative analytics (multiple disciplines): Research and analyze (in support of future decisions). More BI: Monitor, to see when it necessary to decide, plan, or investigate. Yet more BI: Communicate what you’ve learned. DBMS, ETL, etc.: Support the other functions.
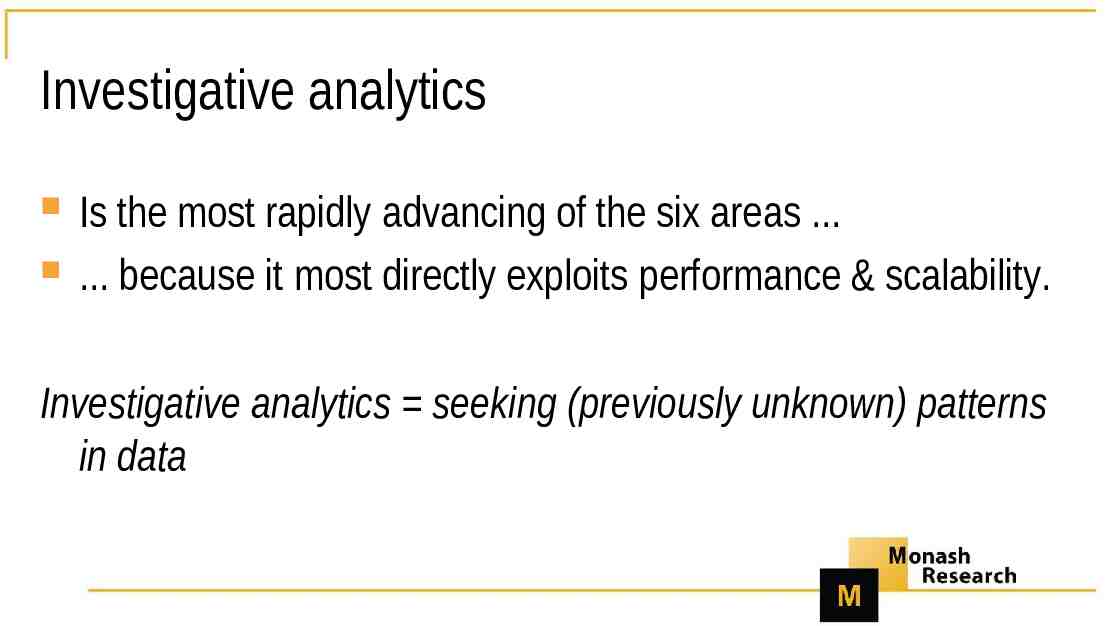
Investigative analytics Is the most rapidly advancing of the six areas . . because it most directly exploits performance & scalability. Investigative analytics seeking (previously unknown) patterns in data
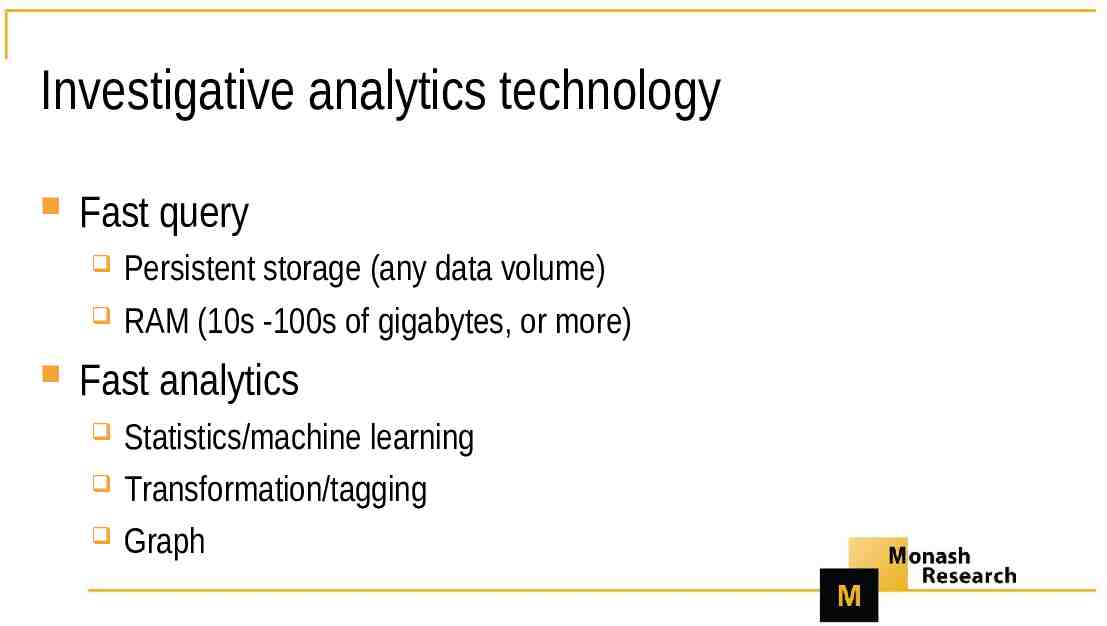
Investigative analytics technology Fast query Persistent storage (any data volume) RAM (10s -100s of gigabytes, or more) Fast analytics Statistics/machine learning Transformation/tagging Graph
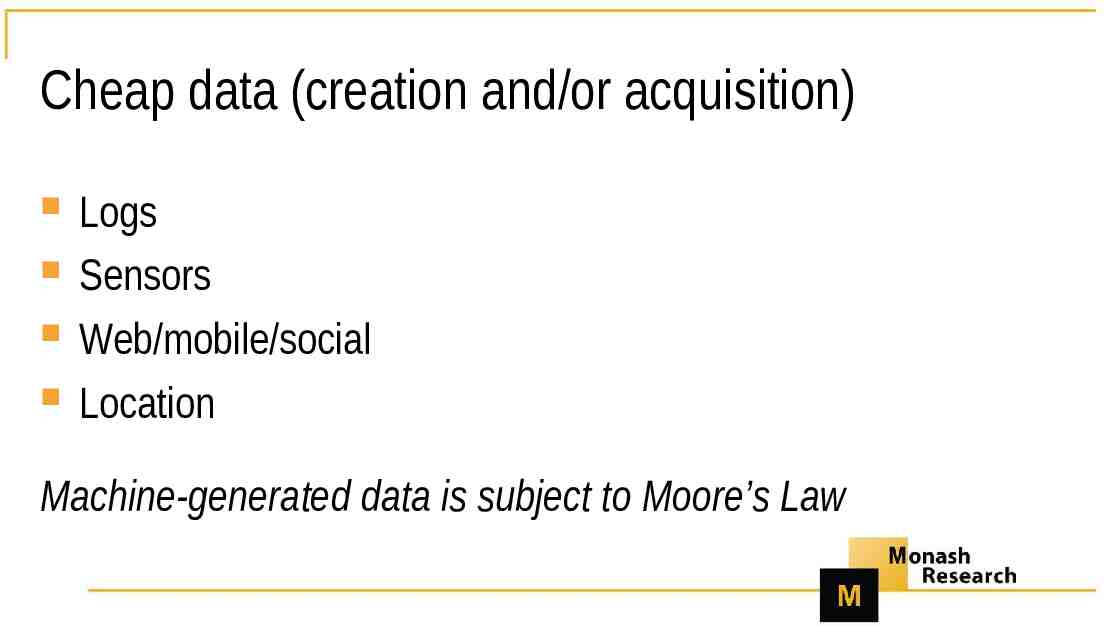
Cheap data (creation and/or acquisition) Logs Sensors Web/mobile/social Location Machine-generated data is subject to Moore’s Law
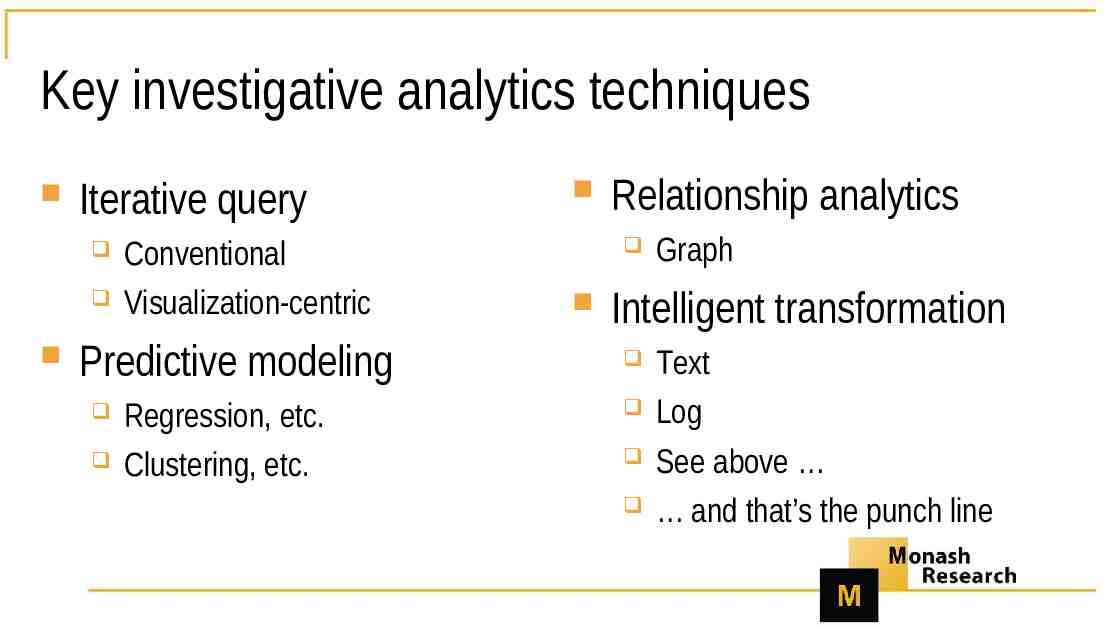
Key investigative analytics techniques Iterative query Conventional Visualization-centric Predictive modeling Regression, etc. Clustering, etc. Relationship analytics Graph Intelligent transformation Text Log See above and that’s the punch line
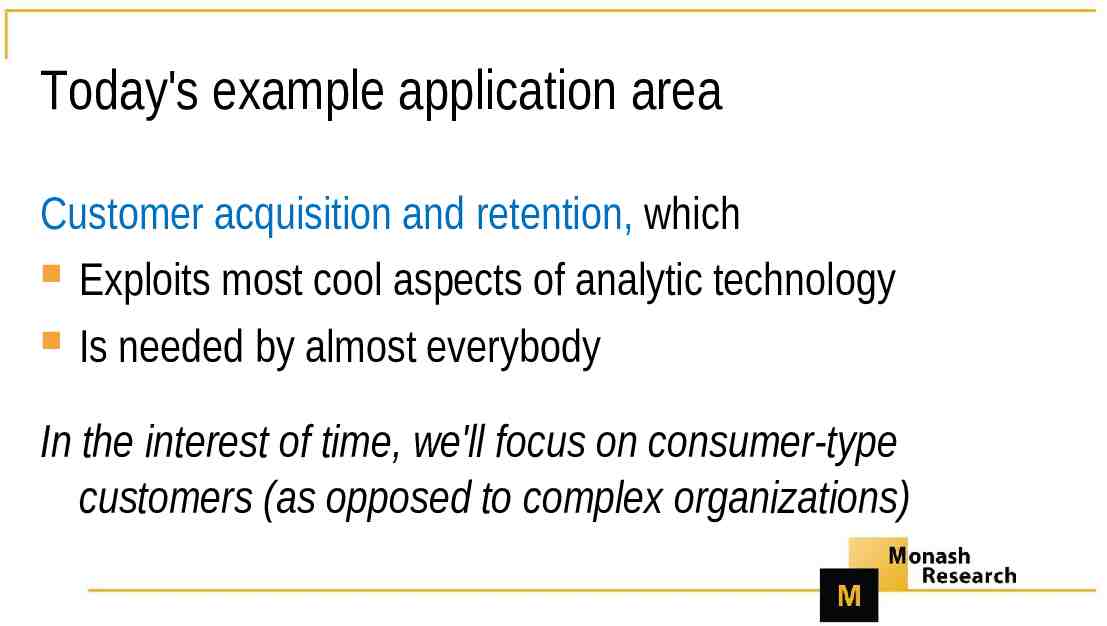
Today's example application area Customer acquisition and retention, which Exploits most cool aspects of analytic technology Is needed by almost everybody In the interest of time, we'll focus on consumer-type customers (as opposed to complex organizations)
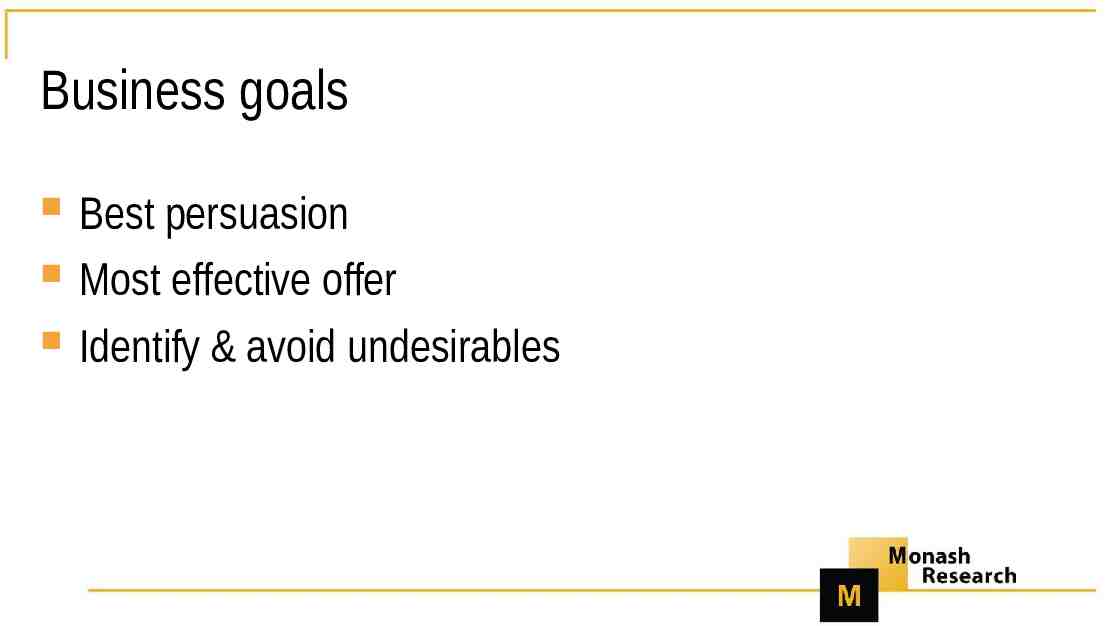
Business goals Best persuasion Most effective offer Identify & avoid undesirables
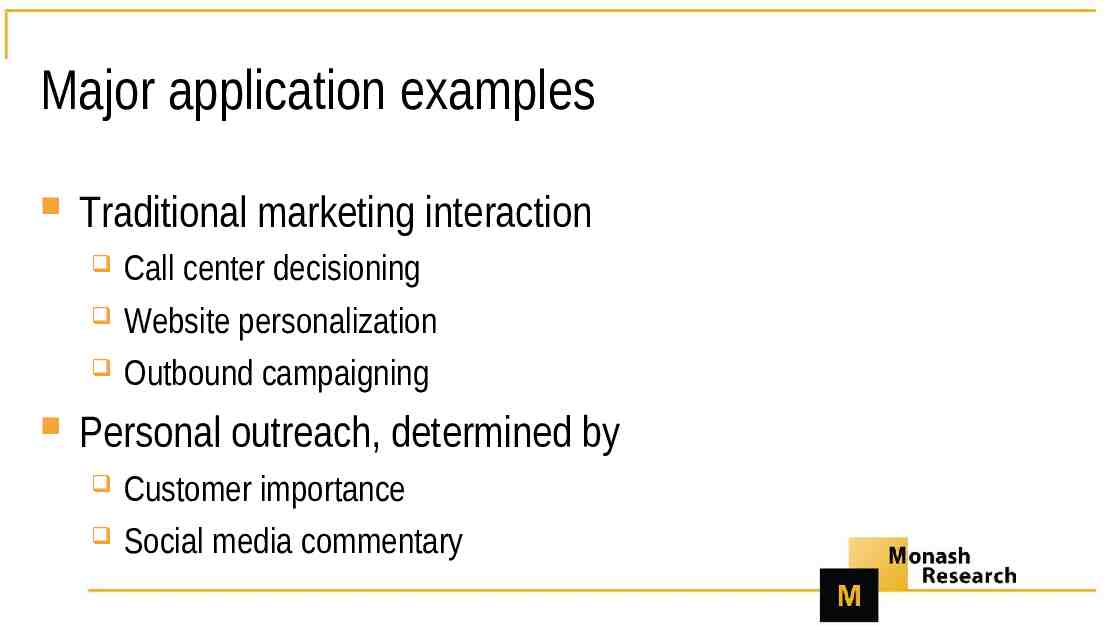
Major application examples Traditional marketing interaction Call center decisioning Website personalization Outbound campaigning Personal outreach, determined by Customer importance Social media commentary
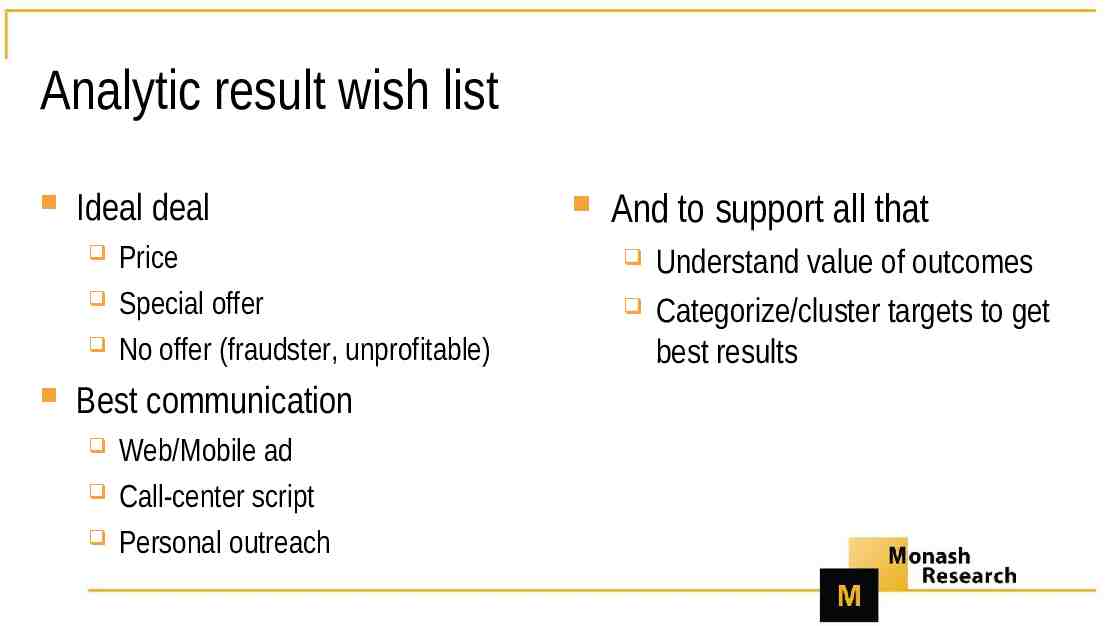
Analytic result wish list Ideal deal Price Special offer No offer (fraudster, unprofitable) Best communication Web/Mobile ad Call-center script Personal outreach And to support all that Understand value of outcomes Categorize/cluster targets to get best results
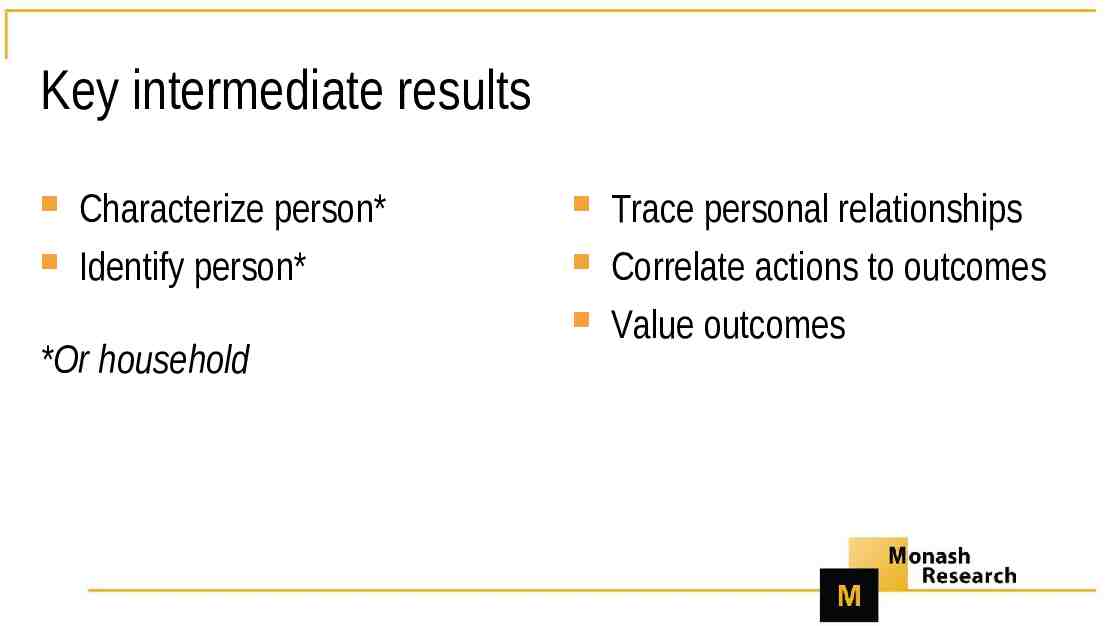
Key intermediate results Characterize person* Identify person* *Or household Trace personal relationships Correlate actions to outcomes Value outcomes
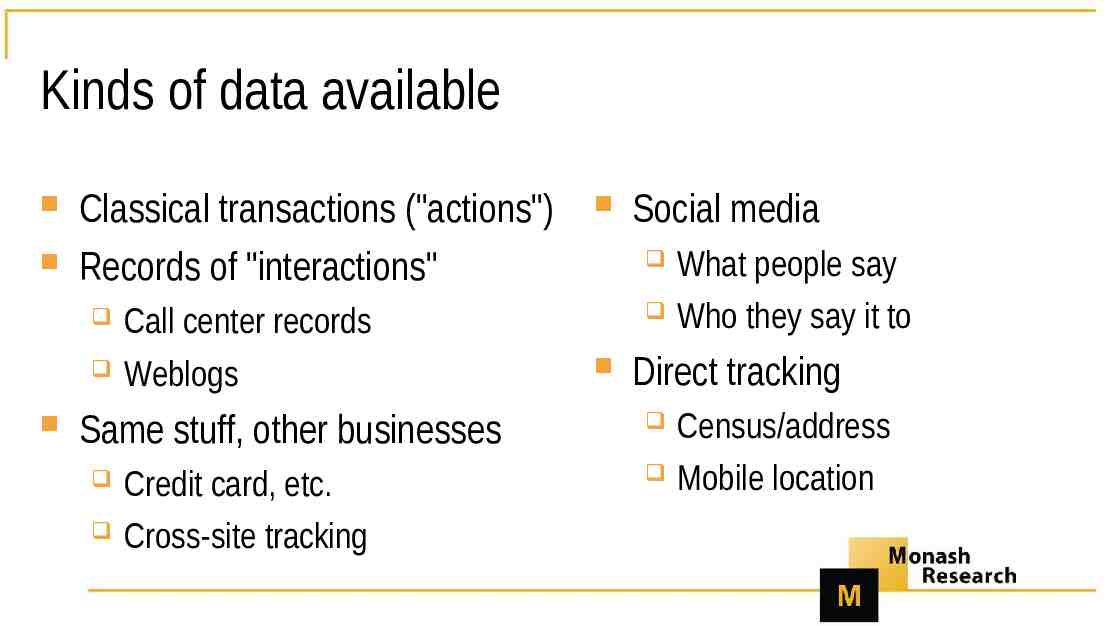
Kinds of data available Classical transactions ("actions") Records of "interactions" Call center records Weblogs Same stuff, other businesses Credit card, etc. Cross-site tracking Social media What people say Who they say it to Direct tracking Census/address Mobile location
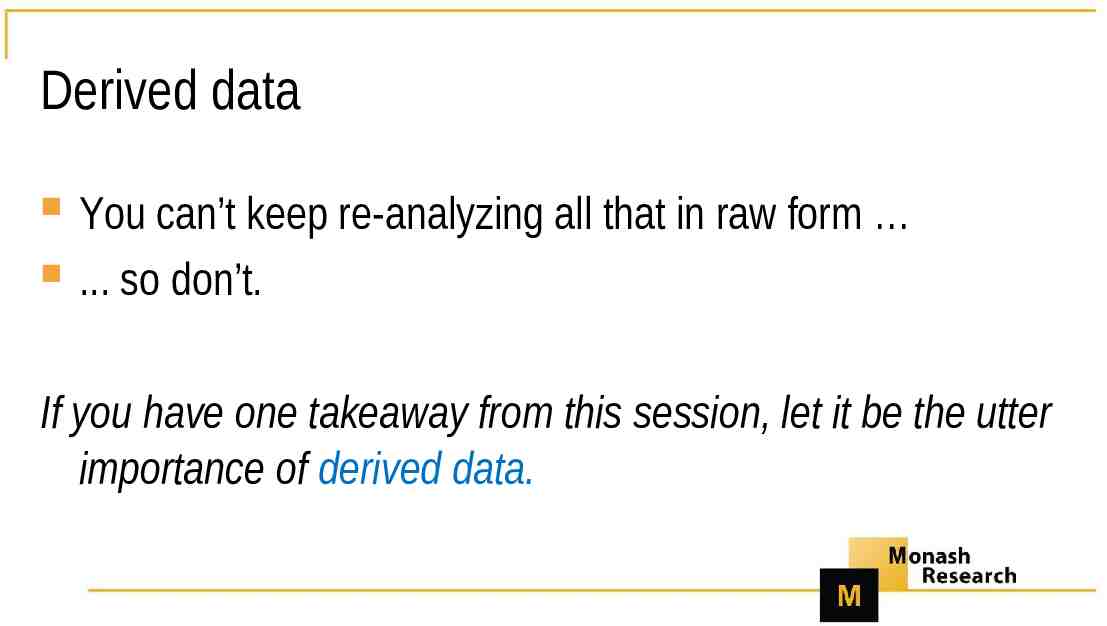
Derived data You can’t keep re-analyzing all that in raw form . so don’t. If you have one takeaway from this session, let it be the utter importance of derived data.
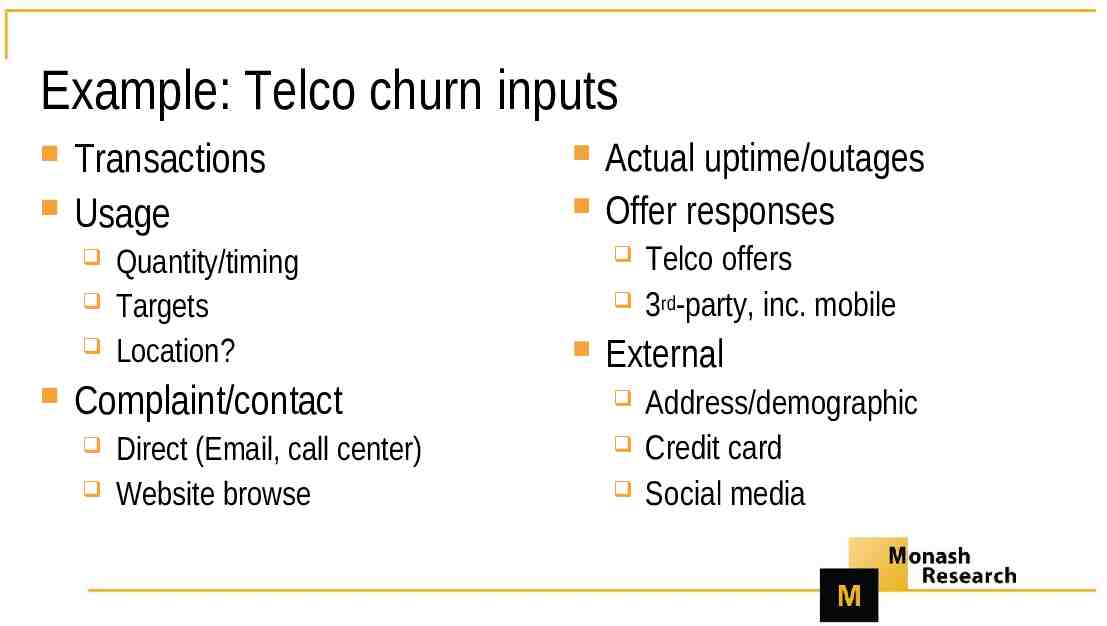
Example: Telco churn inputs Transactions Usage Quantity/timing Targets Location? Complaint/contact Direct (Email, call center) Website browse Actual uptime/outages Offer responses Telco offers 3rd-party, inc. mobile External Address/demographic Credit card Social media
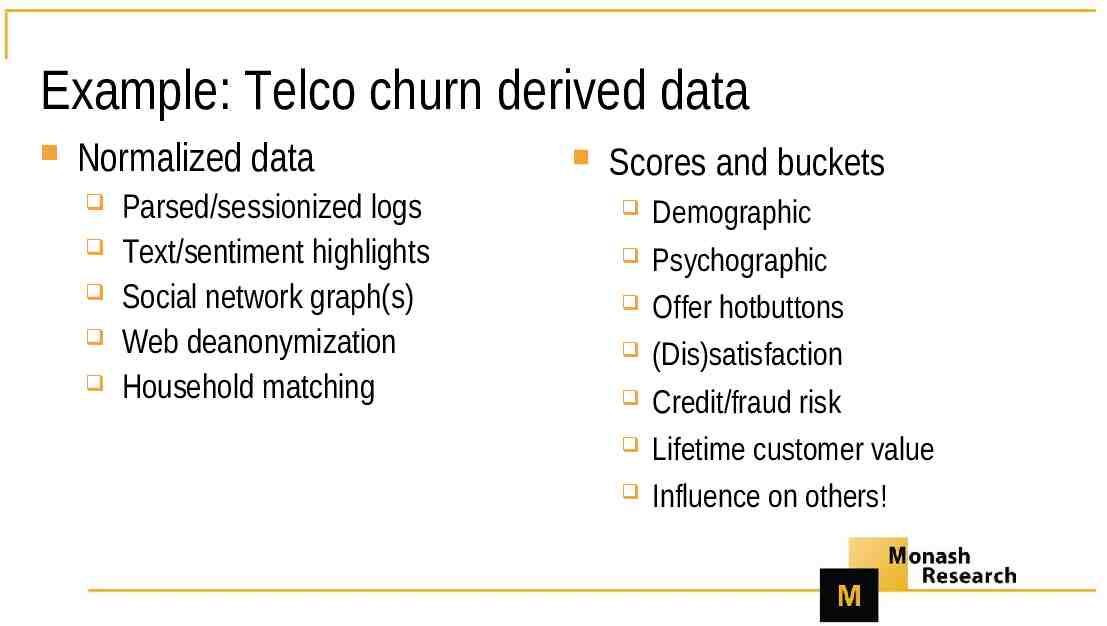
Example: Telco churn derived data Normalized data Parsed/sessionized logs Text/sentiment highlights Social network graph(s) Web deanonymization Household matching Scores and buckets Demographic Psychographic Offer hotbuttons (Dis)satisfaction Credit/fraud risk Lifetime customer value Influence on others!
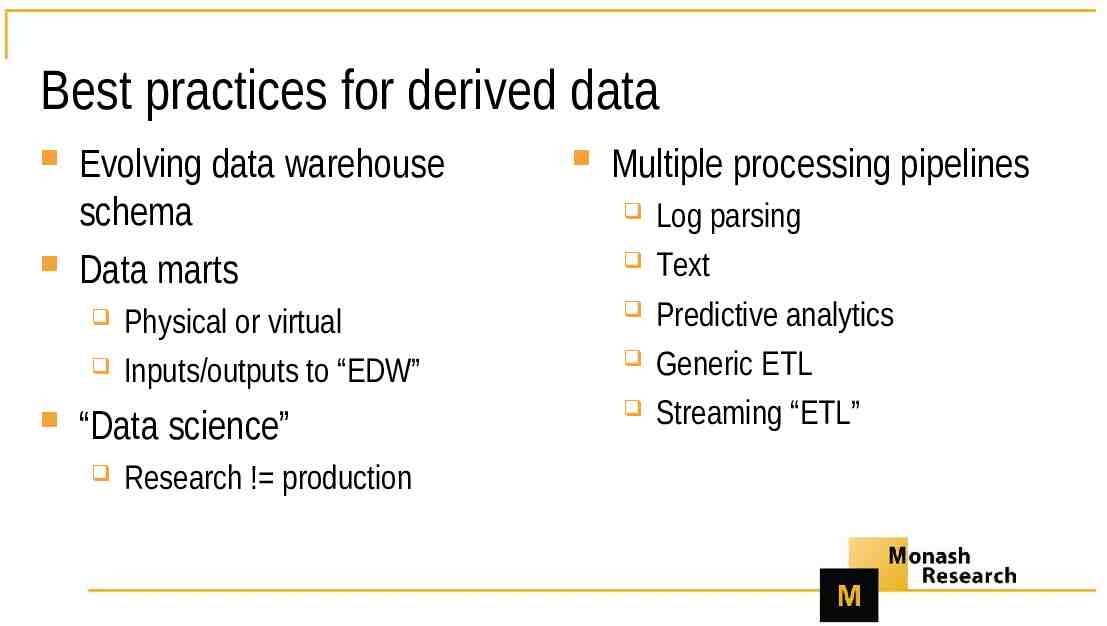
Best practices for derived data Evolving data warehouse schema Data marts Physical or virtual Inputs/outputs to “EDW” “Data science” Research ! production Multiple processing pipelines Log parsing Text Predictive analytics Generic ETL Streaming “ETL”
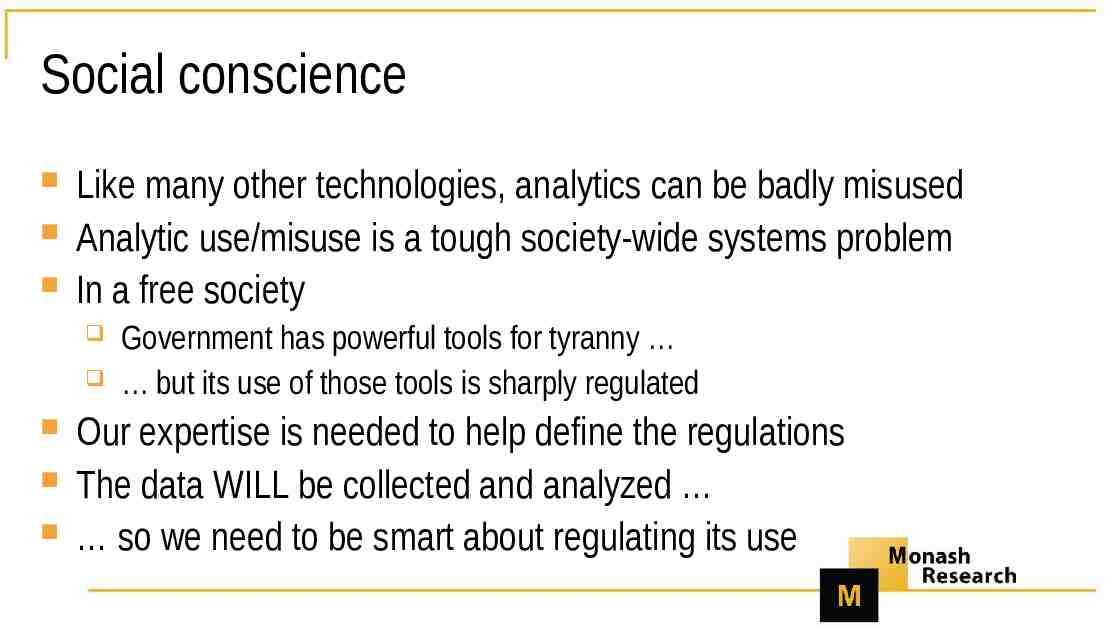
Social conscience Like many other technologies, analytics can be badly misused Analytic use/misuse is a tough society-wide systems problem In a free society Government has powerful tools for tyranny but its use of those tools is sharply regulated Our expertise is needed to help define the regulations The data WILL be collected and analyzed so we need to be smart about regulating its use
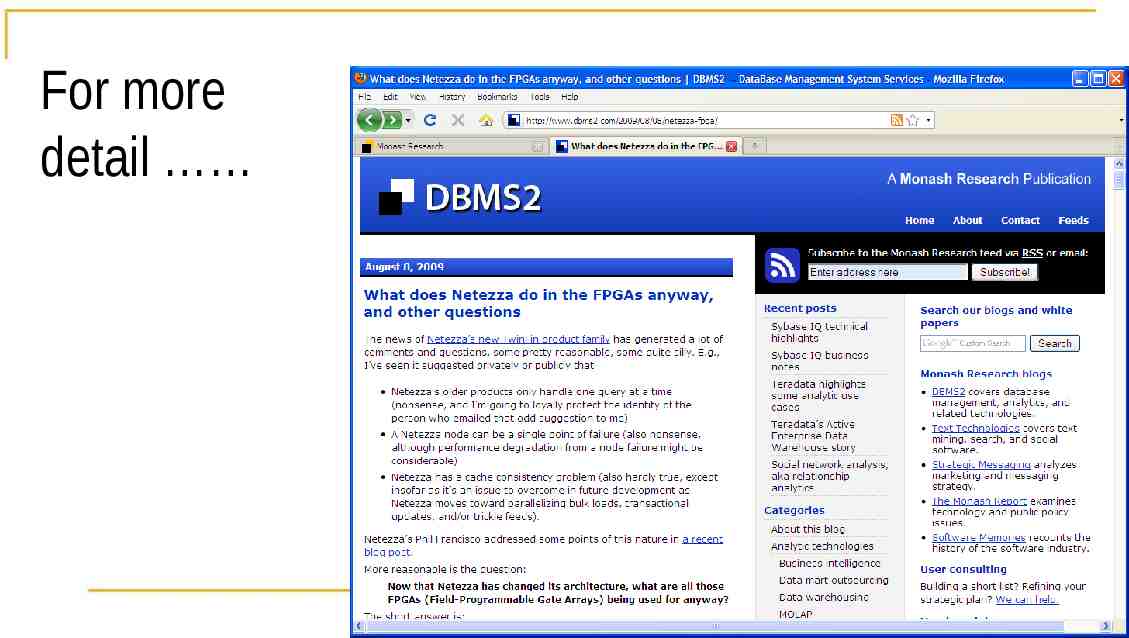
For more detail
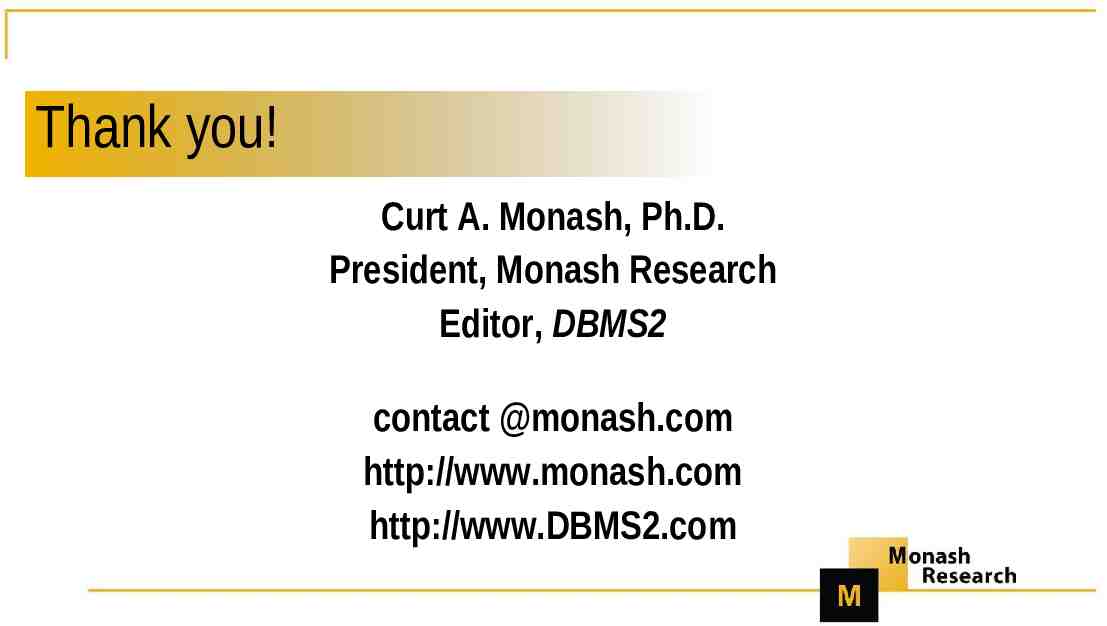
Thank you! Curt A. Monash, Ph.D. President, Monash Research Editor, DBMS2 contact @monash.com http://www.monash.com http://www.DBMS2.com