Data assimilation using a hybrid variational-ensemble Kalman filter
38 Slides5.47 MB

Data assimilation using a hybrid variational-ensemble Kalman filter approach: methodology and recent applications in numerical weather prediction Xuguang Wang School of Meteorology and Center for Analysis and Prediction of Storms, University of Oklahoma, Norman, OK, USA Collaborators: Yongzuo Li, Ming Xue, Dale Barker, Chris Snyder, Tom Hamill Seminar at Environmental Modeling Center, NCEP, Jan 14, 2010

What’s Hybrid? xb xb yo xa VAR VAR EnKF e.g. ETKF xb Xb: forecast Xa: analysis yo: observation VAR: variational (3DVAR, 4DVAR) method EnKF: ensemble Kalman filter ETKF: ensemble transform Kalman filter xa xa xb xb time xb HYBRID Data assimilation part adopts variational framework. Like EnKF, maintain multiple forecasts and analyses cycles. Like EnKF, ensemble forecasts involve in the estimate of background error

Why Hybrid? “Best of both worlds” Hybrid conveniently provides flow-dependent estimate of the background error covariance. Hamill et al. 2006 Wang 2010

Why Hybrid? “Best of both worlds” Studies (Wang et al. 2007b,2008ab, 2009b, Buehner et al. 2009ab) have demonstrated that a hybrid VAR-EnKF can significantly improve upon a standalone VAR system . Studies (Wang et al. 2007b, 2009b) also showed hybrid can improve upon a standalone EnKF for small ensemble size and perhaps because a 4DVAREnKF hybrid can evolve covariance in a higher-dimensional space (Buehner et al. 2009ab). Compared to EnKF, hybrid adopts model space rather than observation space covariance localization, more appropriate for satellite radiance, GPS refractivity assimilation (campbell et al. 2009).

Why Hybrid? “Best of both worlds” Good choice for already having an established VAR and ensemble forecast system (Wang et al. 2007ab, 2009b) Minor changes to the existing operational variational framework Take advantages of existing capability of VAR, e.g., variational data quality control Static covariance model in advanced VAR can provide sophisticated method to reduce sampling errors in ensemble covariance (e.g., anisotropic recursive filter for adaptive covariance localization). Likely to be cost effective with many obs., since minimization does not scale linearly with number of obs. (Wang et al. 2007b). VAR framework provides maximum likelihood solution and thus allows nonGaussian errors (Zupanski 2005). VAR framework can also easily add in dynamic constraint (Kleist et al. 2009). Study (Caya et al. 2005) shows that for radar DA, 4DVAR spins up faster than EnKF, but EnKF is better in later stage of the DA cycles. Hybrid can take advantage of both.

Hybrid Data Assimilation Theory Ensemble covariance is included in the VAR cost function through augmentation of control variables (Lorenc 2003, Buehner 2005, Wang et al. 2007a, 2008a, Wang 2009). J x1' , α 1 J 1 2 J e J o 1 ' ' 1 K T 1 ' T 1 ' 1 1 x1 B x1 2 α T C 1α y o ' Hx ' R 1 y o ' Hx ' 2 2 2 x x α k x ek k 1 Extra term associated with extended control variable Extra increment associated with ensemble B 3DVAR static covariance; R observation error covariance; K ensemble size; C correlation matrix for ensemble covariance localization; x ek kth ensemble perturbation; x1' 3DVAR increment; x ' total (hybrid) increment; y o ' innovation vector; H linearized observation operator; 1 weighting coefficient for static covariance; 2 weighting coefficient for ensemble covariance; α extended control variable.

Hybrid Data Assimilation Theory Wang et al. 2007a proved the solution of the above framework for hybrid is equivalent to that obtained by replacing the original static covariance with the weighted sum of the static covariance and the localized ensemble covariance. It can fit in VAR with preconditioning w.r.t. square root of B (e.g. Wang et al 2008a) and the full B (Wang 2009, submitted).

Hybrid ETKF-3DVAR for WRF Wang et al. 2008ab, Monthly Weather Review (MWR) updated perturbation 1 perturbation 1 member 1 forecast perturbation 2 ETKF update perturbations member 1 forecast member 2 analysis member 2 forecast member 3 analysis member 3 forecast updated perturbation 2 member 2 forecast updated perturbation 3 perturbation 3 member 3 forecast ensemble mean member 1 analysis hybrid update mean data assimilation updated mean forecast

Ensemble generation by Ensemble Transform Kalman filter (ETKF) The ensemble was generated by the ensemble transform Kalman filter (ETKF, Wang and Bishop 2003; Wang et al. 2004, 2007b) Transformation matrix solved from Kalman filter theory X a X b T Initial ensemble perturbation forecast ensemble perturbation

Experiments using the WRF ETKF3DVAR system Tested with various scales and data Month-long large-scale experiment over North America Hurricane IKE, GUSTAV 2008 1-5day track forecast Radar data assimilation: hurricane IKE 2008; Weak continental convection

Month-long NA Experiment Wang et al. 2008ab, MWR WRF domain, observation locations and verification region WRF domain: North America; coarse resolution (Δx 200km; 28 levels) Observation: radiosonde wind and temperature Test period: Jan 2003 Ensemble size: 50 members Verifications: Compare forecasts initialized by the hybrid and 3DVAR analyses.

flow-dependent increments by the hybrid 850mb T increment (k) The hybrid system can provide flow-dependent increments.

12-h wind forecast Why big improvement here? Forecast initialized from analysis by hybrid DA improved over 3DVAR.

flow-dependent cross variable update of moisture field by the hybrid Although no moisture observations were assimilated, hybrid through cross-variable covariance estimated by the ensemble can update moisture field whereas 3DVAR can not. In this case, flow-dependent adjustments produced by the hybrid dried the lower troposphere along the front.

Hurricane track forecast: (Wang 2010 in preparation) IKE, GUSTAV 2008 IKE 14 Tropical storm cat1 13 cat2 cat1 cat3 cat4 12 11 10 9 8 7 WRF Model: Δx 30km; 35 levels Observations: from GTS; all conventional in-situ data plus cloud wind, QuikScat wind. No vortex relocation or bogus. GUSTAV TD Ensemble size: 32 members DA, forecast and verification: 3hrly DA cycling; forecasts after 2-day/3-day spin up for IKE/GUSTAV every day at 00Z. Compare forecasts initialized by WRF 4 3 TD TS cat1 2 cat2 1 cat3 cat431 cat1 30 2928 27 TS

IKE track forecast Averaged track forecast initialized by analyses generated by the hybrid method is more accurate after 2-day lead time. 5 5 4 3 2 1 What happened for the 5-day forecast?

500mb Height analysis at Sep 9 00Z WRF HYBRID Stronger vortex by the hybrid analysis WRF 3DVAR Differences in the west periphery of the ridge

500mb Height forecast at Sep 13 00Z WRF HYBRID Westward track bias by 3DVAR is associated with more westward Ridge. WRF 3DVAR

SLP Increment differences on Sep 8 00z WRF HYBRID increment WRF 3DVAR increment Ensemble spread Ensemble spread suggested major forecast uncertainty around IKE. Increment by the hybrid was mostly at TC. 3DVAR produced large-scale increment.

GUSTAV track forecast Averaged track forecast initialized by analyses generated by the hybrid method is more accurate after 1-day lead time. 5 5 4 3 2 1 What happened for the 4-day forecast?

500mb Height analysis at Aug 31 00Z WRF HYBRID Stronger GUSTAV by the hybrid analysis; asymmetric GUSTAV by 3DVAR WRF 3DVAR Smaller Hanna by the hybrid analysis; asymmetric Hanna by 3DVAR

500mb Height forecast at Sep 3 00Z WRF HYBRID WRF 3DVAR The westward track bias for GUSTAV by WRF 3DVAR appear to be associated with a stronger and bigger Hanna.

SLP Increment differences on Aug 31 00z WRF HYBRID increment WRF 3DVAR increment Ensemble spread Ensemble spread suggested major forecast uncertainty around GUSTAV and Hanna. Increment by the hybrid was mostly at TC. 3DVAR increment was at large-scale.

Hurricane IKE 2008 radar data assimilation experiment (Wang et al. 2010, in preparation) d01 Nested domains: Δx 15km (d01)/5km(d02) Ensemble size: 40 members TS 18h forecast DA and forecast: TS 12h ensemble initialized at 18ZSep12 Cat1 Radar DA at 06ZSep13 DA at 06Z Cat2 18h deterministic forecast from 06ZSep13 No cycling yet Observations: radial velocity from two WSR88D radars (KHGX, KLCH)

Radar data: Vr Preprocessing: Raw data: WSR88D Level II Use wind profile based on RAOB or GFS grid data to create the background De-aliasing using a modified version of Four-Dimensional Doppler Dealiasing Scheme (4DD) (James and Houze, 2001). Thinning: 500m in vertical and 5-10 km in horizontal

Analysis Increment: 850mb wind Hybrid increment is around the eye of IKE, which suggested a stronger IKE than the first guess. 3DVAR increment is smooth and at larger scale without recognizing IKE.

Track and Intensity forecast Track forecast by hybrid was better than WRF 3DVAR and similar to GFS. Hybrid analyzed and predicted a stronger IKE (closer to the best track) than both WRF 3DVAR and GFS.

1h Precipitation forecast Precipitation forecast by the hybrid revealed more detailed structure of the rain-band than 3DVAR.

Radar DA for a weak continental convection case Observations: WSR88D radial wind Ensemble size: 40 members WRF model: x 4km

Wind increment differences: hybrid vs. 3DVAR 3DVAR increments produced a double vortex, which is irrelevant to the background weather Hybrid increments mostly concentrated at the region of active weather (flow-dependent).

Conclusion and discussion WRF Hybrid ETKF-VAR has been developed and applied for various scales assimilating various data and was demonstrated to improve the analyses and subsequent forecasts. Experiments have shown that flow-dependent covariance provided by the ensemble contributed to the better performance by the hybrid. Such flow-dependency is expected to have the biggest impact on data sparse region (TC over open water), less-observed state variables (e.g., moisture), and retrieval of indirectly observed state variables (e.g., radar reflectivity assimilation)

Future work WRF VAR based hybrid: Further improve covariance localization Adding hydrometeors in the analysis for reflectivity assimilation More experiments and detailed diagnostics of IKE/GUSTAV track forecast and radar data assimilation experiments Conduct experiments using WRF 4DVAR-ensemble hybrid to explore if the hybrid is better than 4DVAR and EnKF for mesoscales. GSI based hybrid: Global NWP Inter-comparison of GSI, EnKF, hybrid Best ensemble to be used Advantages in radiance assimilation with model space localization in hybrid Effective utilization of (anisotropic) recursive filter for covariance localization Regional NWP HRRR HWRF NAM/NDAS

References cited Campbell, W. F., C. H. Bishop, D. Hodyss, 2009: Vertical Covariance Localization for Satellite Radiances in Ensemble Kalman Filters. Mon. Wea. Rev., in press. James C. N., and R. A. Houze, 2001: A real—time four-dimensional Doppler dealiasing scheme. J. Atmos. Oceanic Tech., 18, 1647-1683. Lorenc, A. C. 2003: The potential of the ensemble Kalman filter for NWP – a comparison with 4D-VAR. Quart. J. Roy. Meteor. Soc., 129, 3183-3203. Buehner, M., 2005: Ensemble-derived stationary and flow-dependent background-error covariances: evaluation in a quasi-operational NWP setting. Quart. J. Roy. Meteor. Soc., 131, 1013-1043. Wang, X., and C. H. Bishop, 2003: A comparison of breeding and ensemble transform Kalman filter ensemble forecast schemes. J. Atmos. Sci., 60, 1140-1158. Wang, X., C. H. Bishop, and Simon J. Julier, 2004: Which is better, an ensemble of positive-negative pairs or a centered spherical simplex ensemble? Mon. Wea. Rev., 132, 1590-1605. Wang, X., C. Snyder, and T. M. Hamill, 2007a: On the theoretical equivalence of differently proposed ensemble/3D-Var hybrid analysis schemes. Mon. Wea. Rev., 135, 222-227. Wang, X., T. M. Hamill, J. S. Whitaker and C. H. Bishop, 2007b: A comparison of hybrid ensemble transform Kalman filter-OI and ensemble square-root filter analysis schemes. Mon. Wea. Rev., 135, 1055-1076. Wang, X., D. Barker, C. Snyder, T. M. Hamill, 2008a: A hybrid ETKF-3DVar data assimilation scheme for the WRF model. Part I: observing system simulation experiment. Mon. Wea. Rev., 136, 5116-5131. Wang, X., D. Barker, C. Snyder, T. M. Hamill, 2008b: A hybrid ETKF-3DVar data assimilation scheme for the WRF model. Part II: real observation experiments. Mon. Wea. Rev., 136, 5132-5147. Wang, X., T. M. Hamill, J. S. Whitaker, C. H. Bishop, 2009: A comparison of the hybrid and EnSRF analysis schemes in the presence of model error due to unresolved scales. Mon. Wea. Rev., 137, 3219-3232. Wang, X., 2009: Incorporating ensemble covariance in the Gridpoint Statistical Interpolation (GSI) variational minimization: a mathematical framework. Mon. Wea. Rev., submitted. Wang, X., 2010: Hurricane track forecast using the hybrid ETKF-3DVar for WRF, Mon. Wea. Rev., to be submitted. Wang, X., Y. Li and M. Xue, 2010: Radar data assimilation using the hybrid ETKF-3DVar for WRF, Mon. Wea. Rev., to be submitted.

Ensemble Spread: 850mb wind Large ensemble spread at maximum wind speed gradient in the first guess around the eye The ring of the ensemble spread is associated with relatively large innovation, which suggests the spread can distinguish the first guess errors around the eye from other places.
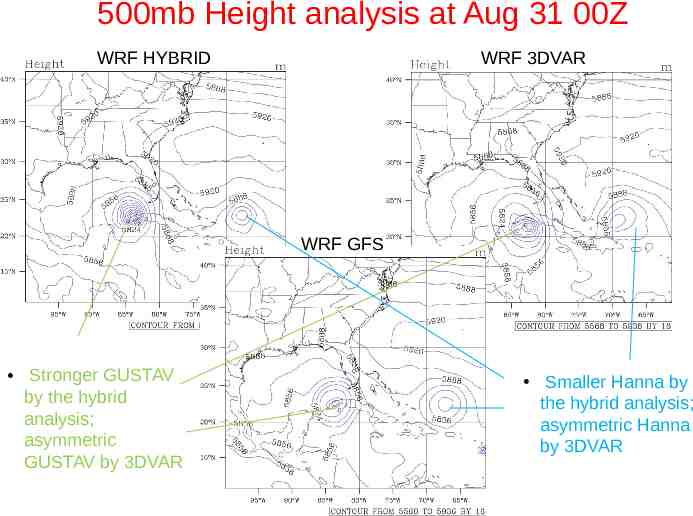
500mb Height analysis at Aug 31 00Z WRF HYBRID WRF 3DVAR WRF GFS Stronger GUSTAV by the hybrid analysis; asymmetric GUSTAV by 3DVAR Smaller Hanna by the hybrid analysis; asymmetric Hanna by 3DVAR

500mb Height forecast at Sep 3 00Z WRF HYBRID WRF 3DVAR WRF GFS The westward by 3DVAR and GFS appear to be associated with a stronger and bigger Hanna. Intensity of Hanna is more close to best track.

What’s data assimilation (DA)? observations model forecast data assimilation analysis DA is a statistical analysis process that combines observations with short-range model forecasts.

Minimum SLP forecast IKE GUSTAV